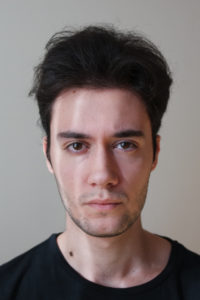
Information
+39 080 596 3843
ti.ab1746771602ilop@1746771602inong1746771602im.al1746771602ocin1746771602
Scopus Researcher Page
Curriculum Vitae (english)
Publications
Nicola MIGNONI
PhD student
Nicola Mignoni received the Bachelor Degree in Management Engineering in July 2019 and the Master Degree in Management Engineering (with specialization in Digital Innovation) in July 2021, both with honours, from the Polytechnic of Bari. He has been a graduate Research Assistant from July to November 2021 at the Decision and Control Laboratory of Department of Electrical and Information Engineering of Polytechnic of Bari.
He is currently working toward the Ph.D. degree in Electrical and Information Engineering, of the same university, under the supervision of Prof. Engr. Mariagrazia Dotoli. His research interests include optimization, game theory and multi-agent systems, with application in control of sustainable Nearly-Zero/Positive Energy Communities.
Publications
2025
- Mignoni, N., Scarabaggio, P., Carli, R. & Dotoli, M. (2025) A Framework for the Automated and Optimal Design of Vertical Lift Modules. IN IEEE Transactions on Systems, Man, and Cybernetics: Systems, .. doi:10.1109/TSMC.2025.3547302
[BibTeX] [Abstract] [Download PDF]To this day, tasks like planning and managing warehouses remain complex. Automated storage and retrieval systems have enhanced warehousing efficiency, yet designing them optimally is still challenging, despite their importance for the efficient operation of the warehouse. This article aims to present a novel framework for automating the optimal design of vertical lift modules (VLMs), focusing on tray types, quantities, and item-tray sector assignments, based on a specified inventory list. The approach accounts for VLM’s physical, manufacturing, and ergonomic constraints to ensure a manufacturable system design. To manage computational complexity, the size of the mixed-integer problem is reduced through an exact clustering of items, sectors, and layouts. The proposed framework is tested through numerical simulations using real data from an Italian VLM manufacturer. © 2013 IEEE.
@ARTICLE{Mignoni2025, author = {Mignoni, Nicola and Scarabaggio, Paolo and Carli, Raffaele and Dotoli, Mariagrazia}, title = {A Framework for the Automated and Optimal Design of Vertical Lift Modules}, year = {2025}, journal = {IEEE Transactions on Systems, Man, and Cybernetics: Systems}, doi = {10.1109/TSMC.2025.3547302}, url = {https://www.scopus.com/inward/record.uri?eid=2-s2.0-105000390110&doi=10.1109%2fTSMC.2025.3547302&partnerID=40&md5=8e3e2b43920755886d79bd2e6a3e8eb7}, abstract = {To this day, tasks like planning and managing warehouses remain complex. Automated storage and retrieval systems have enhanced warehousing efficiency, yet designing them optimally is still challenging, despite their importance for the efficient operation of the warehouse. This article aims to present a novel framework for automating the optimal design of vertical lift modules (VLMs), focusing on tray types, quantities, and item-tray sector assignments, based on a specified inventory list. The approach accounts for VLM's physical, manufacturing, and ergonomic constraints to ensure a manufacturable system design. To manage computational complexity, the size of the mixed-integer problem is reduced through an exact clustering of items, sectors, and layouts. The proposed framework is tested through numerical simulations using real data from an Italian VLM manufacturer. © 2013 IEEE.}, author_keywords = {Automatic storage and retrieval systems; combinatorial assignment; logistics; mixed-integer optimization; vertical lift modules (VLMs)}, type = {Article}, publication_stage = {Article in press}, source = {Scopus}, note = {Cited by: 0} }
2024
- Mignoni, N., Scarabaggio, P., Carli, R. & Dotoli, M. (2024) A Markowitz Optimization Approach for Automating the Italian Research Quality Monitoring and Evaluation IN IEEE International Conference on Automation Science and Engineering., 1741 – 1746. doi:10.1109/CASE59546.2024.10711557
[BibTeX] [Abstract] [Download PDF]This paper presents a selection-supporting framework which can help research institutions to optimally and automatically pool the research products for research quality assessment programs, with a specific focus on the Italian evaluation process (VQR). After providing a mathematical description of the VQR exercise at the institutional level, we formulate the robust optimization problem which yields the optimal pair of research products and associated authors. We show how such a formulation quickly becomes unpractical, due to combinatorial issues, and propose a novel Markowitz-based alternative approach, which preserves computational feasibility and effectiveness. We strive to propose a reusable framework, not too tightly connected to the ruleset of the current VQR session (2020-2024). Finally, we validate the proposed framework on a synthetic set of parameters, which mimics a medium-sized research institution, with the aim of checking the computational feasibility of the proposed Markowitz-based variants. © 2024 IEEE.
@CONFERENCE{Mignoni20241741, author = {Mignoni, Nicola and Scarabaggio, Paolo and Carli, Raffaele and Dotoli, Mariagrazia}, title = {A Markowitz Optimization Approach for Automating the Italian Research Quality Monitoring and Evaluation}, year = {2024}, journal = {IEEE International Conference on Automation Science and Engineering}, pages = {1741 – 1746}, doi = {10.1109/CASE59546.2024.10711557}, url = {https://www.scopus.com/inward/record.uri?eid=2-s2.0-85208240014&doi=10.1109%2fCASE59546.2024.10711557&partnerID=40&md5=f248874234f038dc9b0af1c54eed84c1}, abstract = {This paper presents a selection-supporting framework which can help research institutions to optimally and automatically pool the research products for research quality assessment programs, with a specific focus on the Italian evaluation process (VQR). After providing a mathematical description of the VQR exercise at the institutional level, we formulate the robust optimization problem which yields the optimal pair of research products and associated authors. We show how such a formulation quickly becomes unpractical, due to combinatorial issues, and propose a novel Markowitz-based alternative approach, which preserves computational feasibility and effectiveness. We strive to propose a reusable framework, not too tightly connected to the ruleset of the current VQR session (2020-2024). Finally, we validate the proposed framework on a synthetic set of parameters, which mimics a medium-sized research institution, with the aim of checking the computational feasibility of the proposed Markowitz-based variants. © 2024 IEEE.}, type = {Conference paper}, publication_stage = {Final}, source = {Scopus}, note = {Cited by: 0} }
- Scarabaggio, P., Mignoni, N., Carli, R. & Dotoli, M. (2024) Equilibrium Seeking in Learning-Based Noncooperative Nash Games IN Proceedings of the IEEE Conference on Decision and Control., 210 – 215. doi:10.1109/CDC56724.2024.10886614
[BibTeX] [Abstract] [Download PDF]Traditionally, based on convexity, multi-agent decision-making models can hardly handle scenarios where agents’ cost functions defy this assumption, which is specifically required to ensure the existence of several equilibrium concepts. More recently, the advent of machine learning (ML), with its inherent non-convexity, has changed the conventional approach of pursuing convexity at all costs. This paper explores and integrates the robustness of game theoretic frameworks in managing conflicts among agents with the capacity of ML approaches, such as deep neural networks (DNNs), to capture complex agent behaviors. Specifically, we employ feed-forward DNNs to characterize agents’ best response actions rather than modeling their goals with convex functions. We introduce a technical assumption on the weight of the DNN to establish the existence and uniqueness of Nash equilibria and present two distributed algorithms based on fixed-point iterations for their computation. Finally, we demonstrate the practical application of our framework to a noncooperative community of smart energy users under a dynamic time-of-use energy pricing scheme. © 2024 IEEE.
@CONFERENCE{Scarabaggio2024210, author = {Scarabaggio, Paolo and Mignoni, Nicola and Carli, Raffaele and Dotoli, Mariagrazia}, title = {Equilibrium Seeking in Learning-Based Noncooperative Nash Games}, year = {2024}, journal = {Proceedings of the IEEE Conference on Decision and Control}, pages = {210 – 215}, doi = {10.1109/CDC56724.2024.10886614}, url = {https://www.scopus.com/inward/record.uri?eid=2-s2.0-86000515374&doi=10.1109%2fCDC56724.2024.10886614&partnerID=40&md5=f0bab8c0cd8fec781bc22c981b8eab20}, abstract = {Traditionally, based on convexity, multi-agent decision-making models can hardly handle scenarios where agents' cost functions defy this assumption, which is specifically required to ensure the existence of several equilibrium concepts. More recently, the advent of machine learning (ML), with its inherent non-convexity, has changed the conventional approach of pursuing convexity at all costs. This paper explores and integrates the robustness of game theoretic frameworks in managing conflicts among agents with the capacity of ML approaches, such as deep neural networks (DNNs), to capture complex agent behaviors. Specifically, we employ feed-forward DNNs to characterize agents' best response actions rather than modeling their goals with convex functions. We introduce a technical assumption on the weight of the DNN to establish the existence and uniqueness of Nash equilibria and present two distributed algorithms based on fixed-point iterations for their computation. Finally, we demonstrate the practical application of our framework to a noncooperative community of smart energy users under a dynamic time-of-use energy pricing scheme. © 2024 IEEE.}, type = {Conference paper}, publication_stage = {Final}, source = {Scopus}, note = {Cited by: 0} }
- Scarabaggio, P., Mignoni, N., Jantzen, J., Carli, R. & Dotoli, M. (2024) Model Predictive Control with Recursive Multi-step Input Convex Lipschitz Neural Networks: an Application to Smart Buildings IN Conference Proceedings – IEEE International Conference on Systems, Man and Cybernetics., 4005 – 4010. doi:10.1109/SMC54092.2024.10831606
[BibTeX] [Abstract] [Download PDF]Model Predictive Control (MPC) is an optimal control technique that employs a dynamic model of the controlled process and an optimization algorithm to determine the control strategy. Nevertheless, the cost and effort required to create and maintain dynamical models are often high, and solving the resulting optimal control problem can be computationally complex. In recent years, data-driven modeling has become an attractive alternative to approximate the behavior of dynamical systems, with the aim of alleviating these issues. However, using such models for model-based control can be challenging due to their typically nonlinear and nonconvex nature. To address these issues, we propose a recursive multi-step learning-based dynamical modeling framework to capture the temporal behavior of dynamic systems. We take advantage of Input Convex Lipschitz Neural Networks, which are explicitly designed to be convex and continuous with respect to their in-puts. We further show that these mathematical proprieties hold in a multi-step dynamical modeling framework. The proposed approach is evaluated in a real-life MPC experiment conducted in a smart building in the Samso Marina, Denmark. We show that the proposed approach keeps the internal temperature within comfort constraints while minimizing heating/cooling energy consumption. © 2024 IEEE.
@CONFERENCE{Scarabaggio20244005, author = {Scarabaggio, Paolo and Mignoni, Nicola and Jantzen, Jan and Carli, Raffaele and Dotoli, Mariagrazia}, title = {Model Predictive Control with Recursive Multi-step Input Convex Lipschitz Neural Networks: an Application to Smart Buildings}, year = {2024}, journal = {Conference Proceedings - IEEE International Conference on Systems, Man and Cybernetics}, pages = {4005 – 4010}, doi = {10.1109/SMC54092.2024.10831606}, url = {https://www.scopus.com/inward/record.uri?eid=2-s2.0-85217858869&doi=10.1109%2fSMC54092.2024.10831606&partnerID=40&md5=33bc3afa8c718f5d9ceac4544c8b7302}, abstract = {Model Predictive Control (MPC) is an optimal control technique that employs a dynamic model of the controlled process and an optimization algorithm to determine the control strategy. Nevertheless, the cost and effort required to create and maintain dynamical models are often high, and solving the resulting optimal control problem can be computationally complex. In recent years, data-driven modeling has become an attractive alternative to approximate the behavior of dynamical systems, with the aim of alleviating these issues. However, using such models for model-based control can be challenging due to their typically nonlinear and nonconvex nature. To address these issues, we propose a recursive multi-step learning-based dynamical modeling framework to capture the temporal behavior of dynamic systems. We take advantage of Input Convex Lipschitz Neural Networks, which are explicitly designed to be convex and continuous with respect to their in-puts. We further show that these mathematical proprieties hold in a multi-step dynamical modeling framework. The proposed approach is evaluated in a real-life MPC experiment conducted in a smart building in the Samso Marina, Denmark. We show that the proposed approach keeps the internal temperature within comfort constraints while minimizing heating/cooling energy consumption. © 2024 IEEE.}, author_keywords = {Building Automation; Convex Optimization; Model Predictive Control; Neural Networks; Supervised Learning}, type = {Conference paper}, publication_stage = {Final}, source = {Scopus}, note = {Cited by: 0} }
- Mignoni, N., Carli, R. & Dotoli, M. (2024) Optimal Decision Strategies for the Generalized Cuckoo Card Game. IN IEEE Transactions on Games, 16.185 – 194. doi:10.1109/TG.2023.3239795
[BibTeX] [Abstract] [Download PDF]Cuckoo is a popular card game, which originated in France during the 15th century, and then, spread throughout Europe, where it is currently well-known under distinct names and with different variants. Cuckoo is an imperfect information game-of-chance, which makes the research regarding its optimal strategies determination interesting. The rules are simple: each player receives a covered card from the dealer; starting from the player at the dealer’s left, each player looks at its own card and decides whether to exchange it with the player to their left, or keep it; the dealer plays at last and, if it decides to exchange card, it draws a random one from the remaining deck; the player(s) with the lowest valued card lose(s) the round. We formulate the gameplay mathematically and provide an analysis of the optimal decision policies. Different card decks can be used for this game, e.g., the standard 52-card deck or the Italian 40-card deck. We generalize the decision model for an arbitrary number of decks’ cards, suites, and players. Finally, through numerical simulations, we compare the determined optimal decision strategy against different benchmarks, showing that the strategy outperforms the random and naive policies and approaches the performance of the ideal oracle. © 2018 IEEE.
@ARTICLE{Mignoni2024185, author = {Mignoni, Nicola and Carli, Raffaele and Dotoli, Mariagrazia}, title = {Optimal Decision Strategies for the Generalized Cuckoo Card Game}, year = {2024}, journal = {IEEE Transactions on Games}, volume = {16}, number = {1}, pages = {185 – 194}, doi = {10.1109/TG.2023.3239795}, url = {https://www.scopus.com/inward/record.uri?eid=2-s2.0-85147283813&doi=10.1109%2fTG.2023.3239795&partnerID=40&md5=3913f77d7fa5cb1c10485170111b23d2}, abstract = {Cuckoo is a popular card game, which originated in France during the 15th century, and then, spread throughout Europe, where it is currently well-known under distinct names and with different variants. Cuckoo is an imperfect information game-of-chance, which makes the research regarding its optimal strategies determination interesting. The rules are simple: each player receives a covered card from the dealer; starting from the player at the dealer's left, each player looks at its own card and decides whether to exchange it with the player to their left, or keep it; the dealer plays at last and, if it decides to exchange card, it draws a random one from the remaining deck; the player(s) with the lowest valued card lose(s) the round. We formulate the gameplay mathematically and provide an analysis of the optimal decision policies. Different card decks can be used for this game, e.g., the standard 52-card deck or the Italian 40-card deck. We generalize the decision model for an arbitrary number of decks' cards, suites, and players. Finally, through numerical simulations, we compare the determined optimal decision strategy against different benchmarks, showing that the strategy outperforms the random and naive policies and approaches the performance of the ideal oracle. © 2018 IEEE.}, author_keywords = {Card games; games of chance; optimal decision strategy; optimization}, type = {Article}, publication_stage = {Final}, source = {Scopus}, note = {Cited by: 1; All Open Access, Green Open Access, Hybrid Gold Open Access} }
- Mignoni, N., Scarabaggio, P., Caselli, S. M., Carli, R. & Dotoli, M. (2024) Generalized Nash Equilibrium Seeking for Crop Mix Selection in Sustainable Agriculture IN 2024 7th IEEE International Humanitarian Technologies Conference, IHTC 2024.. doi:10.1109/IHTC61819.2024.10855039
[BibTeX] [Abstract] [Download PDF]Since diversifying crop selection can significantly optimize resources, increase resilience to climate change, and contribute to sustainable development, decision-making tools are crucial to help farmers identify optimal crop cultivation strategies. This paper proposes a novel optimization framework for crop mix selection under the non-cooperative game-theoretical perspective, i.e., assuming that the agents (e.g., farmers and agriculture companies) behave selfishly. Such a perspective naturally arises when multiple agents are competing for shared resources, such as water, land, and carbon emissions quotas. First, we illustrate the system model, comprising both local and global resource constraints that the crop mix strategy of all agents must abide by. Then, we characterize the Generalized Nash Equilibrium problem stemming from such a formulation, providing an iterative equilibrium-seeking approach based on the consolidated operator splitting framework. Finally, we numerically test the proposed framework using real-world data. © 2024 IEEE.
@CONFERENCE{Mignoni2024, author = {Mignoni, Nicola and Scarabaggio, Paolo and Caselli, Sonia Marina and Carli, Raffaele and Dotoli, Mariagrazia}, title = {Generalized Nash Equilibrium Seeking for Crop Mix Selection in Sustainable Agriculture}, year = {2024}, journal = {2024 7th IEEE International Humanitarian Technologies Conference, IHTC 2024}, doi = {10.1109/IHTC61819.2024.10855039}, url = {https://www.scopus.com/inward/record.uri?eid=2-s2.0-85217838518&doi=10.1109%2fIHTC61819.2024.10855039&partnerID=40&md5=5b71c043f8e65d34514cc668aedb80d0}, abstract = {Since diversifying crop selection can significantly optimize resources, increase resilience to climate change, and contribute to sustainable development, decision-making tools are crucial to help farmers identify optimal crop cultivation strategies. This paper proposes a novel optimization framework for crop mix selection under the non-cooperative game-theoretical perspective, i.e., assuming that the agents (e.g., farmers and agriculture companies) behave selfishly. Such a perspective naturally arises when multiple agents are competing for shared resources, such as water, land, and carbon emissions quotas. First, we illustrate the system model, comprising both local and global resource constraints that the crop mix strategy of all agents must abide by. Then, we characterize the Generalized Nash Equilibrium problem stemming from such a formulation, providing an iterative equilibrium-seeking approach based on the consolidated operator splitting framework. Finally, we numerically test the proposed framework using real-world data. © 2024 IEEE.}, author_keywords = {Agriculture 4.0; crop mix; game theory; Nash equilibrium seeking; optimization; sustainability}, type = {Conference paper}, publication_stage = {Final}, source = {Scopus}, note = {Cited by: 0} }
- Mignoni, N., Martinez-Piazuelo, J., Carli, R., Ocampo-Martinez, C., Quijano, N. & Dotoli, M. (2024) A Game-Theoretical Control Framework for Transactive Energy Trading in Energy Communities IN 2024 European Control Conference, ECC 2024., 786 – 791. doi:10.23919/ECC64448.2024.10591157
[BibTeX] [Abstract] [Download PDF]Under the umbrella of non-cooperative game theory, we formulate a transactive energy framework to model and control energy communities comprised of heterogeneous agents including (yet not limited to) prosumers, energy storage systems, and energy retailers. The underlying control task is defined as a generalized Nash equilibrium problem (GNEP), which must be solved in a distributed fashion. To solve the GNEP, we formulate a Gauss-Seidel-type alternating direction method of multipliers algorithm, which is guaranteed to converge under strongly monotone pseudo-gradient mappings. As such, we provide sufficient conditions on the private cost and energy pricing functions of the community members, so that the strong monotonicity of the overall pseudo-gradient is ensured. Finally, the proposed framework and the effectiveness of the solution method are illustrated through a numerical simulation. © 2024 EUCA.
@CONFERENCE{Mignoni2024786, author = {Mignoni, Nicola and Martinez-Piazuelo, Juan and Carli, Raffaele and Ocampo-Martinez, Carlos and Quijano, Nicanor and Dotoli, Mariagrazia}, title = {A Game-Theoretical Control Framework for Transactive Energy Trading in Energy Communities}, year = {2024}, journal = {2024 European Control Conference, ECC 2024}, pages = {786 – 791}, doi = {10.23919/ECC64448.2024.10591157}, url = {https://www.scopus.com/inward/record.uri?eid=2-s2.0-85200567031&doi=10.23919%2fECC64448.2024.10591157&partnerID=40&md5=940a89b8b7a2b8a90d241be8bd443e4b}, abstract = {Under the umbrella of non-cooperative game theory, we formulate a transactive energy framework to model and control energy communities comprised of heterogeneous agents including (yet not limited to) prosumers, energy storage systems, and energy retailers. The underlying control task is defined as a generalized Nash equilibrium problem (GNEP), which must be solved in a distributed fashion. To solve the GNEP, we formulate a Gauss-Seidel-type alternating direction method of multipliers algorithm, which is guaranteed to converge under strongly monotone pseudo-gradient mappings. As such, we provide sufficient conditions on the private cost and energy pricing functions of the community members, so that the strong monotonicity of the overall pseudo-gradient is ensured. Finally, the proposed framework and the effectiveness of the solution method are illustrated through a numerical simulation. © 2024 EUCA.}, type = {Conference paper}, publication_stage = {Final}, source = {Scopus}, note = {Cited by: 0} }
- Mignoni, N., Scarabaggio, P., Carli, R. & Dotoli, M. (2024) A Compact Convex Quadratically Constrained Formulation for a Class of Delivery Schedule Problems IN Proceedings of the IEEE Conference on Decision and Control., 8403 – 8408. doi:10.1109/CDC56724.2024.10885832
[BibTeX] [Abstract] [Download PDF]In this paper, we present a novel and efficient formulation of a common class of delivery schedule optimization problems. We define the overall model structure, which includes warehouses, clients, and service stations that can be visited by delivery agents, as well as the operational constraints the delivery process must abide, aiming at maximizing the number of delivered orders. We first provide a linear problem formulation, constituting the main baseline; hence, we construct an exact and more compact quadratically constrained version, which reduces the number of binary variables involved. Furthermore, we validate the proposed approach on a realistic numerical example, showing how less computational resources are needed, with respect to the baseline version, without excessively slowing down the convergence towards the optimum. © 2024 IEEE.
@CONFERENCE{Mignoni20248403, author = {Mignoni, Nicola and Scarabaggio, Paolo and Carli, Raffaele and Dotoli, Mariagrazia}, title = {A Compact Convex Quadratically Constrained Formulation for a Class of Delivery Schedule Problems}, year = {2024}, journal = {Proceedings of the IEEE Conference on Decision and Control}, pages = {8403 – 8408}, doi = {10.1109/CDC56724.2024.10885832}, url = {https://www.scopus.com/inward/record.uri?eid=2-s2.0-86000578121&doi=10.1109%2fCDC56724.2024.10885832&partnerID=40&md5=49e4d01c4d8ce4ffe9e0878227230f75}, abstract = {In this paper, we present a novel and efficient formulation of a common class of delivery schedule optimization problems. We define the overall model structure, which includes warehouses, clients, and service stations that can be visited by delivery agents, as well as the operational constraints the delivery process must abide, aiming at maximizing the number of delivered orders. We first provide a linear problem formulation, constituting the main baseline; hence, we construct an exact and more compact quadratically constrained version, which reduces the number of binary variables involved. Furthermore, we validate the proposed approach on a realistic numerical example, showing how less computational resources are needed, with respect to the baseline version, without excessively slowing down the convergence towards the optimum. © 2024 IEEE.}, type = {Conference paper}, publication_stage = {Final}, source = {Scopus}, note = {Cited by: 0} }
2023
- Mignoni, N., Carli, R. & Dotoli, M. (2023) Layout Optimization for Photovoltaic Panels in Solar Power Plants via a MINLP Approach. IN IEEE Transactions on Automation Science and Engineering, .1–14. doi:10.1109/TASE.2023.3322786
[BibTeX] [Abstract] [Download PDF]Photovoltaic (PV) technology is one of the most popular means of renewable generation, whose applications range from commercial and residential buildings to industrial facilities and grid infrastructures. The problem of determining a suitable layout for the PV arrays, on a given deployment region, is generally non-trivial and has a crucial importance in the planning phase of solar plants design and development. In this paper, we provide a mixed integer non-linear programming formulation of the PV arrays’ layout problem. First, we define the astronomical and geometrical models, considering crucial factors such as self-shadowing and irradiance variability, depending on the geographical position of the solar plant and yearly time window. Subsequently, we formalize the mathematical optimization problem, whose constraints’ set is characterized by non-convexities. In order to propose a computationally tractable approach, we provide a tight parametrized convex relaxation. The resulting optimization resolution procedure is tested numerically, using realistic data, and benchmarked against the traditional global resolution approach, showing that the proposed methodology yields near-optimal solutions in lower computational time.
Note to Practitioners —The paper is motivated by the need for efficient algorithmic procedures which can yield near-optimal solutions to the PV arrays layout problem. Due to the strong non-convexity of even simple instances, the existing methods heavily rely on global or stochastic solvers, which are computationally demanding, both in terms of resources and run-time. Our approach acts as a baseline, from which practitioners can derive more elaborate instances, by suitably modifying both the objective function and/or the constraints. In fact, we focus on the minimum set of necessary geometrical (e.g., arrays position model), astronomical (e.g., irradiance variation), and operational (e.g., power requirements) constraints which make the overall problem hard. The Appendices provide a guideline for suitably choosing the optimization parameters. All data and simulation code are available on a public repository at: https://github.com/nicomignoni/pvlayout.git. Authors@ARTICLE{Mignoni20231, author = {Mignoni, Nicola and Carli, Raffaele and Dotoli, Mariagrazia}, title = {Layout Optimization for Photovoltaic Panels in Solar Power Plants via a MINLP Approach}, year = {2023}, journal = {IEEE Transactions on Automation Science and Engineering}, pages = {1–14}, doi = {10.1109/TASE.2023.3322786}, url = {https://www.scopus.com/inward/record.uri?eid=2-s2.0-85181574361&doi=10.1109%2fTASE.2023.3322786&partnerID=40&md5=81a2752bb7efb922f17af8b437e4693b}, abstract = {Photovoltaic (PV) technology is one of the most popular means of renewable generation, whose applications range from commercial and residential buildings to industrial facilities and grid infrastructures. The problem of determining a suitable layout for the PV arrays, on a given deployment region, is generally non-trivial and has a crucial importance in the planning phase of solar plants design and development. In this paper, we provide a mixed integer non-linear programming formulation of the PV arrays’ layout problem. First, we define the astronomical and geometrical models, considering crucial factors such as self-shadowing and irradiance variability, depending on the geographical position of the solar plant and yearly time window. Subsequently, we formalize the mathematical optimization problem, whose constraints’ set is characterized by non-convexities. In order to propose a computationally tractable approach, we provide a tight parametrized convex relaxation. The resulting optimization resolution procedure is tested numerically, using realistic data, and benchmarked against the traditional global resolution approach, showing that the proposed methodology yields near-optimal solutions in lower computational time.
Note to Practitioners —The paper is motivated by the need for efficient algorithmic procedures which can yield near-optimal solutions to the PV arrays layout problem. Due to the strong non-convexity of even simple instances, the existing methods heavily rely on global or stochastic solvers, which are computationally demanding, both in terms of resources and run-time. Our approach acts as a baseline, from which practitioners can derive more elaborate instances, by suitably modifying both the objective function and/or the constraints. In fact, we focus on the minimum set of necessary geometrical (e.g., arrays position model), astronomical (e.g., irradiance variation), and operational (e.g., power requirements) constraints which make the overall problem hard. The Appendices provide a guideline for suitably choosing the optimization parameters. All data and simulation code are available on a public repository at: https://github.com/nicomignoni/pvlayout.git. Authors}, author_keywords = {Azimuth; convexification; Layout; Mathematical models; mixed integer non-linear programming; non-convex optimization; Observers; Optimization; parametrization; Photovoltaic; Photovoltaic systems; solar array layout; solar power plants; Sun}, type = {Article}, publication_stage = {Article in press}, source = {Scopus}, note = {Cited by: 3; All Open Access, Green Open Access, Hybrid Gold Open Access} } - Mignoni, N., Carli, R. & Dotoli, M. (2023) A Noncooperative Stochastic Rolling Horizon Control Framework for V1G and V2B Scheduling in Energy Communities IN 2023 European Control Conference, ECC 2023.. doi:10.23919/ECC57647.2023.10178202
[BibTeX] [Abstract] [Download PDF]In this paper, we propose a novel control strategy for the optimal scheduling of an energy community constituted by prosumers and equipped with unidirectional vehicle-to-grid (V1G) and vehicle-to-building (V2B) capabilities. In particular, V2B services are provided by long-term parked electric vehicles (EVs) thus acting as temporary storage systems by prosumers, which in turn offer the V1G service to EVs provisionally plugged into charging stations. To tackle the stochastic nature of the framework, we assume that EVs communicate their parking and recharging time distribution to prosumers, allowing them to improve their energy allocation. Prosumers and EVs interact in a rolling horizon control framework with the aim of achieving an agreement on their operating strategies. The resulting control problem is formulated as a generalized Nash equilibrium problem, addressed through the variational inequality theory, and solved in a distributed fashion leveraging on the accelerated distributed augmented Lagrangian method (ADALM). The convergence and effectiveness of the proposed approach are validated through numerical simulations under realistic scenarios. © 2023 EUCA.
@CONFERENCE{Mignoni2023, author = {Mignoni, Nicola and Carli, Raffaele and Dotoli, Mariagrazia}, title = {A Noncooperative Stochastic Rolling Horizon Control Framework for V1G and V2B Scheduling in Energy Communities}, year = {2023}, journal = {2023 European Control Conference, ECC 2023}, doi = {10.23919/ECC57647.2023.10178202}, url = {https://www.scopus.com/inward/record.uri?eid=2-s2.0-85166479889&doi=10.23919%2fECC57647.2023.10178202&partnerID=40&md5=e34ca4551ecbe785858f9937e0c0a38c}, abstract = {In this paper, we propose a novel control strategy for the optimal scheduling of an energy community constituted by prosumers and equipped with unidirectional vehicle-to-grid (V1G) and vehicle-to-building (V2B) capabilities. In particular, V2B services are provided by long-term parked electric vehicles (EVs) thus acting as temporary storage systems by prosumers, which in turn offer the V1G service to EVs provisionally plugged into charging stations. To tackle the stochastic nature of the framework, we assume that EVs communicate their parking and recharging time distribution to prosumers, allowing them to improve their energy allocation. Prosumers and EVs interact in a rolling horizon control framework with the aim of achieving an agreement on their operating strategies. The resulting control problem is formulated as a generalized Nash equilibrium problem, addressed through the variational inequality theory, and solved in a distributed fashion leveraging on the accelerated distributed augmented Lagrangian method (ADALM). The convergence and effectiveness of the proposed approach are validated through numerical simulations under realistic scenarios. © 2023 EUCA.}, type = {Conference paper}, publication_stage = {Final}, source = {Scopus}, note = {Cited by: 0} }
- Mignoni, N., Carli, R. & Dotoli, M. (2023) Distributed Noncooperative MPC for Energy Scheduling of Charging and Trading Electric Vehicles in Energy Communities. IN IEEE Transactions on Control Systems Technology, 31.2159 – 2172. doi:10.1109/TCST.2023.3291549
[BibTeX] [Abstract] [Download PDF]In this article, we propose a novel control strategy for the optimal scheduling of an energy community (EC) constituted by prosumers and equipped with unidirectional vehicle-to-grid (V1G) and vehicle-to-building (V2B) capabilities. In particular, V2B services are provided by long-term parked electric vehicles (EVs), used as temporary storage systems by prosumers, who in turn offer the V1G service to EVs provisionally plugged into charging stations. To tackle the stochastic nature of the framework, we assume that EVs communicate their parking and recharging time distribution to prosumers, allowing them to improve the energy allocation process. Acting as selfish agents, prosumers and EVs interact in a rolling horizon control framework with the aim of achieving an agreement on their operating strategies. The resulting control problem is formulated as a generalized Nash equilibrium (NE) problem, addressed through the variational inequality (VI) theory, and solved in a distributed fashion leveraging on the accelerated distributed augmented Lagrangian method (ADALM), showing sufficient conditions for guaranteeing convergence. The proposed model predictive control (MPC) approach is validated through numerical simulations under realistic scenarios. © 1993-2012 IEEE.
@ARTICLE{Mignoni20232159, author = {Mignoni, Nicola and Carli, Raffaele and Dotoli, Mariagrazia}, title = {Distributed Noncooperative MPC for Energy Scheduling of Charging and Trading Electric Vehicles in Energy Communities}, year = {2023}, journal = {IEEE Transactions on Control Systems Technology}, volume = {31}, number = {5}, pages = {2159 – 2172}, doi = {10.1109/TCST.2023.3291549}, url = {https://www.scopus.com/inward/record.uri?eid=2-s2.0-85165268827&doi=10.1109%2fTCST.2023.3291549&partnerID=40&md5=a1747e82483205009749a103f96f0f12}, abstract = {In this article, we propose a novel control strategy for the optimal scheduling of an energy community (EC) constituted by prosumers and equipped with unidirectional vehicle-to-grid (V1G) and vehicle-to-building (V2B) capabilities. In particular, V2B services are provided by long-term parked electric vehicles (EVs), used as temporary storage systems by prosumers, who in turn offer the V1G service to EVs provisionally plugged into charging stations. To tackle the stochastic nature of the framework, we assume that EVs communicate their parking and recharging time distribution to prosumers, allowing them to improve the energy allocation process. Acting as selfish agents, prosumers and EVs interact in a rolling horizon control framework with the aim of achieving an agreement on their operating strategies. The resulting control problem is formulated as a generalized Nash equilibrium (NE) problem, addressed through the variational inequality (VI) theory, and solved in a distributed fashion leveraging on the accelerated distributed augmented Lagrangian method (ADALM), showing sufficient conditions for guaranteeing convergence. The proposed model predictive control (MPC) approach is validated through numerical simulations under realistic scenarios. © 1993-2012 IEEE.}, author_keywords = {Distributed optimization; electric vehicles (EVs); energy communities (ECs); game theory; model predictive control (MPC); unilateral vehicle-to-grid (V1G); vehicle-to-building (V2B)}, type = {Article}, publication_stage = {Final}, source = {Scopus}, note = {Cited by: 17; All Open Access, Green Open Access, Hybrid Gold Open Access} }
- Mignoni, N., Scarabaggio, P., Carli, R. & Dotoli, M. (2023) Control frameworks for transactive energy storage services in energy communities. IN Control Engineering Practice, 130.. doi:10.1016/j.conengprac.2022.105364
[BibTeX] [Abstract] [Download PDF]Recently, the decreasing cost of storage technologies and the emergence of economy-driven mechanisms for energy exchange are contributing to the spread of energy communities. In this context, this paper aims at defining innovative transactive control frameworks for energy communities equipped with independent service-oriented energy storage systems. The addressed control problem consists in optimally scheduling the energy activities of a group of prosumers, characterized by their own demand and renewable generation, and a group of energy storage service providers, able to store the prosumers’ energy surplus and, subsequently, release it upon a fee payment. We propose two novel resolution algorithms based on a game theoretical control formulation, a coordinated and an uncoordinated one, which can be alternatively used depending on the underlying communication architecture of the grid. The two proposed approaches are validated through numerical simulations on realistic scenarios. Results show that the use of a particular framework does not alter fairness, at least at the community level, i.e., no participant in the groups of prosumers or providers can strongly benefit from changing its strategy while compromising others’ welfare. Lastly, the approaches are compared with a centralized control method showing better computational results. © 2022 Elsevier Ltd
@ARTICLE{Mignoni2023, author = {Mignoni, Nicola and Scarabaggio, Paolo and Carli, Raffaele and Dotoli, Mariagrazia}, title = {Control frameworks for transactive energy storage services in energy communities}, year = {2023}, journal = {Control Engineering Practice}, volume = {130}, doi = {10.1016/j.conengprac.2022.105364}, url = {https://www.scopus.com/inward/record.uri?eid=2-s2.0-85140312671&doi=10.1016%2fj.conengprac.2022.105364&partnerID=40&md5=c2f86f25172d9c67e24d1f09c9b54689}, abstract = {Recently, the decreasing cost of storage technologies and the emergence of economy-driven mechanisms for energy exchange are contributing to the spread of energy communities. In this context, this paper aims at defining innovative transactive control frameworks for energy communities equipped with independent service-oriented energy storage systems. The addressed control problem consists in optimally scheduling the energy activities of a group of prosumers, characterized by their own demand and renewable generation, and a group of energy storage service providers, able to store the prosumers’ energy surplus and, subsequently, release it upon a fee payment. We propose two novel resolution algorithms based on a game theoretical control formulation, a coordinated and an uncoordinated one, which can be alternatively used depending on the underlying communication architecture of the grid. The two proposed approaches are validated through numerical simulations on realistic scenarios. Results show that the use of a particular framework does not alter fairness, at least at the community level, i.e., no participant in the groups of prosumers or providers can strongly benefit from changing its strategy while compromising others’ welfare. Lastly, the approaches are compared with a centralized control method showing better computational results. © 2022 Elsevier Ltd}, author_keywords = {Distributed control; Energy communities; Energy storage systems; Game theory; Smart grids; Transactive control; Transactive energy management}, type = {Article}, publication_stage = {Final}, source = {Scopus}, note = {Cited by: 38; All Open Access, Green Open Access} }
- Mignoni, N., Carli, R. & Dotoli, M. (2023) An Optimization Tool for Displacing Photovoltaic Arrays in Polygonal Areas IN EUROCON 2023 – 20th International Conference on Smart Technologies, Proceedings., 573 – 578. doi:10.1109/EUROCON56442.2023.10198934
[BibTeX] [Abstract] [Download PDF]In this paper, we discuss the problem of optimally designing the layout of a given number of photovoltaic arrays on a flat polygonal surface, in order to maximize a suitable objective function, e.g., the total generated energy. This means finding their optimal position, azimuth and tilt. The considered problem becomes non-trivial when considering effects such as irradiance variability and self-shadowing. We first provide a description of the system model and the associated optimization problem, showing how the resulting formulation presents non-convexities. Then, we provide a tight parametrized convex relaxation, which is computationally tractable and for which optimality conditions hold. We provide numerical simulations using realistic data, showing how the proposed methodology yields near-optimal solutions in lower computational time with respect to the traditional global resolution approach. © 2023 IEEE.
@CONFERENCE{Mignoni2023573, author = {Mignoni, Nicola and Carli, Raffaele and Dotoli, Mariagrazia}, title = {An Optimization Tool for Displacing Photovoltaic Arrays in Polygonal Areas}, year = {2023}, journal = {EUROCON 2023 - 20th International Conference on Smart Technologies, Proceedings}, pages = {573 – 578}, doi = {10.1109/EUROCON56442.2023.10198934}, url = {https://www.scopus.com/inward/record.uri?eid=2-s2.0-85168705720&doi=10.1109%2fEUROCON56442.2023.10198934&partnerID=40&md5=918aa0710a8540e5ce9ea625b0a385d4}, abstract = {In this paper, we discuss the problem of optimally designing the layout of a given number of photovoltaic arrays on a flat polygonal surface, in order to maximize a suitable objective function, e.g., the total generated energy. This means finding their optimal position, azimuth and tilt. The considered problem becomes non-trivial when considering effects such as irradiance variability and self-shadowing. We first provide a description of the system model and the associated optimization problem, showing how the resulting formulation presents non-convexities. Then, we provide a tight parametrized convex relaxation, which is computationally tractable and for which optimality conditions hold. We provide numerical simulations using realistic data, showing how the proposed methodology yields near-optimal solutions in lower computational time with respect to the traditional global resolution approach. © 2023 IEEE.}, type = {Conference paper}, publication_stage = {Final}, source = {Scopus}, note = {Cited by: 1} }
2022
- Mignoni, N., Scarabaggio, P., Carli, R. & Dotoli, M. (2022) Game Theoretical Control Frameworks for Multiple Energy Storage Services in Energy Communities IN 2022 8th International Conference on Control, Decision and Information Technologies, CoDIT 2022., 1580 – 1585. doi:10.1109/CoDIT55151.2022.9804087
[BibTeX] [Abstract] [Download PDF]In the last decade, distributed energy generation and storage have significantly contributed to the widespread of energy communities. In this context, we propose an energy community model constituted by prosumers, characterized by their own demand and renewable generation, and service-oriented energy storage providers, able to store energy surplus and release it upon a fee payment. We address the problem of optimally schedule the energy flows in the community, with the final goal of making the prosumers’ energy supply more efficient, while creating a sustainable and profitable business model for storage providers. The proposed resolution algorithms are based on decentralized and distributed game theoretical control schemes. These approaches are mathematically formulated and then effectively validated and compared with a centralized method through numerical simulations on realistic scenarios. © 2022 IEEE.
@CONFERENCE{Mignoni20221580, author = {Mignoni, Nicola and Scarabaggio, Paolo and Carli, Raffaele and Dotoli, Mariagrazia}, title = {Game Theoretical Control Frameworks for Multiple Energy Storage Services in Energy Communities}, year = {2022}, journal = {2022 8th International Conference on Control, Decision and Information Technologies, CoDIT 2022}, pages = {1580 – 1585}, doi = {10.1109/CoDIT55151.2022.9804087}, url = {https://www.scopus.com/inward/record.uri?eid=2-s2.0-85134303591&doi=10.1109%2fCoDIT55151.2022.9804087&partnerID=40&md5=c5c686570c9998d9639b765af7b24245}, abstract = {In the last decade, distributed energy generation and storage have significantly contributed to the widespread of energy communities. In this context, we propose an energy community model constituted by prosumers, characterized by their own demand and renewable generation, and service-oriented energy storage providers, able to store energy surplus and release it upon a fee payment. We address the problem of optimally schedule the energy flows in the community, with the final goal of making the prosumers' energy supply more efficient, while creating a sustainable and profitable business model for storage providers. The proposed resolution algorithms are based on decentralized and distributed game theoretical control schemes. These approaches are mathematically formulated and then effectively validated and compared with a centralized method through numerical simulations on realistic scenarios. © 2022 IEEE.}, type = {Conference paper}, publication_stage = {Final}, source = {Scopus}, note = {Cited by: 1} }