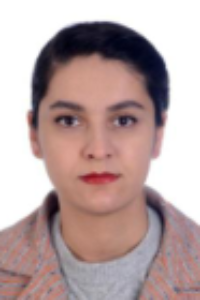
Information
+39 080 596 3843
ti.ab1748761792ilop.1748761792dhp@i1748761792nahgo1748761792nirak1748761792sa.s1748761792
Curriculum Vitae (english)
Publications
Saba Askari Noghani
PhD student
Saba Askari Noghani received her BSc degree in Medical Engineering from Azad University with a great GPA of 4.8. She had been to the University of Minnesota, USA, for a period of six months as a visiting researcher in 2018. She graduated from the Ferdowsi University of Mashhad as the first-ranked student with a master’s degree in Electrical Engineering-Control with honors. She is currently working toward the Ph.D. degree in the Department of Electrical and Information Engineering of Politecnico di Bari with scholarship funded by DAUSY under the supervision of Prof. Engr. Mariagrazia Dotoli.
Her research interest includes modelling, optimization, game theory, and control of complex multi-agent systems.
Publications
2024
- Noghani, S. A., Scarabaggio, P., Carli, R. & Dotoli, M. (2024) Solar-Powered Electric Vehicles into V2G-Capable Smart Parking Infrastructure for Enhanced Energy Efficiency IN 10th 2024 International Conference on Control, Decision and Information Technologies, CoDIT 2024., 575 – 580. doi:10.1109/CoDIT62066.2024.10708524
[BibTeX] [Abstract] [Download PDF]This paper introduces a novel framework for integrating solar-powered electric vehicles (SPEVs) into smart parking infrastructures, primarily focusing on optimizing energy utilization. The proposed framework relies on Model Predictive Control (MPC) to ensure efficient power flow management within smart parking infrastructures. Notably, the paper emphasizes the constraints necessary to ensure the safety and optimal performance of SPEVs and their charging requirements. Results show the effectiveness of the proposed approach, not only in preventing energy management issues but also in substantially reducing reliance on energy procurement from the grid. This integrated system contributes to a more sustainable and cost-effective energy ecosystem, representing a noteworthy advancement in electric mobility infrastructure. © 2024 IEEE.
@CONFERENCE{Noghani2024575, author = {Noghani, Saba Askari and Scarabaggio, Paolo and Carli, Raffaele and Dotoli, Mariagrazia}, title = {Solar-Powered Electric Vehicles into V2G-Capable Smart Parking Infrastructure for Enhanced Energy Efficiency}, year = {2024}, journal = {10th 2024 International Conference on Control, Decision and Information Technologies, CoDIT 2024}, pages = {575 – 580}, doi = {10.1109/CoDIT62066.2024.10708524}, url = {https://www.scopus.com/inward/record.uri?eid=2-s2.0-85208219403&doi=10.1109%2fCoDIT62066.2024.10708524&partnerID=40&md5=bf6191f3ce28a0a3f86fb1105d8412c1}, abstract = {This paper introduces a novel framework for integrating solar-powered electric vehicles (SPEVs) into smart parking infrastructures, primarily focusing on optimizing energy utilization. The proposed framework relies on Model Predictive Control (MPC) to ensure efficient power flow management within smart parking infrastructures. Notably, the paper emphasizes the constraints necessary to ensure the safety and optimal performance of SPEVs and their charging requirements. Results show the effectiveness of the proposed approach, not only in preventing energy management issues but also in substantially reducing reliance on energy procurement from the grid. This integrated system contributes to a more sustainable and cost-effective energy ecosystem, representing a noteworthy advancement in electric mobility infrastructure. © 2024 IEEE.}, author_keywords = {energy management; model predictive control; renewable energy; solar-powered vehicles}, type = {Conference paper}, publication_stage = {Final}, source = {Scopus}, note = {Cited by: 1} }
- Noghani, S. A., Scarabaggio, P., Carli, R. & Dotoli, M. (2024) Noncooperative Control of Energy Communities through Learning-based Response Dynamics IN IEEE International Conference on Automation Science and Engineering., 2732 – 2737. doi:10.1109/CASE59546.2024.10711353
[BibTeX] [Abstract] [Download PDF]With the growing availability of data, learning-based distributed energy management is emerging as a viable and efficient alternative to traditional model-based schemes. In this context, we propose a novel game-theoretic learning-based method for the distributed control of energy communities. In particular, we consider a community that includes several prosumers equipped with a renewable energy source and an energy storage system. The scheduling of energy activities of all prosumers is formulated as a noncooperative game. Nevertheless, unlike the state-of-the-art, where an optimization problem is typically defined to model the behavior of each prosumer, we approximate each prosumer response strategy using a neural network. We propose a distributed algorithm based on the well-known Banach-Picard iteration to efficiently seek for an equilibrium of the game. Lastly, the convergence and effectiveness of the proposed approach are validated through numerical simulations under different realistic scenarios. © 2024 IEEE.
@CONFERENCE{Noghani20242732, author = {Noghani, Saba Askari and Scarabaggio, Paolo and Carli, Raffaele and Dotoli, Mariagrazia}, title = {Noncooperative Control of Energy Communities through Learning-based Response Dynamics}, year = {2024}, journal = {IEEE International Conference on Automation Science and Engineering}, pages = {2732 – 2737}, doi = {10.1109/CASE59546.2024.10711353}, url = {https://www.scopus.com/inward/record.uri?eid=2-s2.0-85208262627&doi=10.1109%2fCASE59546.2024.10711353&partnerID=40&md5=e074e4dacc838b87babe72ee8773baf3}, abstract = {With the growing availability of data, learning-based distributed energy management is emerging as a viable and efficient alternative to traditional model-based schemes. In this context, we propose a novel game-theoretic learning-based method for the distributed control of energy communities. In particular, we consider a community that includes several prosumers equipped with a renewable energy source and an energy storage system. The scheduling of energy activities of all prosumers is formulated as a noncooperative game. Nevertheless, unlike the state-of-the-art, where an optimization problem is typically defined to model the behavior of each prosumer, we approximate each prosumer response strategy using a neural network. We propose a distributed algorithm based on the well-known Banach-Picard iteration to efficiently seek for an equilibrium of the game. Lastly, the convergence and effectiveness of the proposed approach are validated through numerical simulations under different realistic scenarios. © 2024 IEEE.}, author_keywords = {energy management; game theory; learning-based; optimization; Renewable energy}, type = {Conference paper}, publication_stage = {Final}, source = {Scopus}, note = {Cited by: 0} }