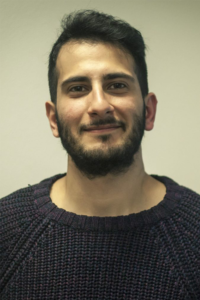
Information
“L. Salvatore” building – 3° floor – room 308
+39 080 596 3065
ti.ab1732190386ilop@1732190386oigga1732190386barac1732190386s.olo1732190386ap1732190386
Institutional page
Scopus Researcher Page
Curriculum Vitae (english)
Curriculum Vitae (italian)
Pubblicazioni
Courses
Analisi e Simulazione dei Sistemi
Fondamenti di Automatica
Game Theory for Controlling Autonomous Systems
Paolo SCARABAGGIO
Assistant Professor
Paolo Scarabaggio received a Ph.D. in Electrical and Information Engineering from Politecnico di Bari, Italy.
He is currently an assistant professor (RTDA) at the Decision and Control Laboratory of Politecnico di Bari. In 2019, he was a visiting student at the Delft Center for Systems and Control, Technical University of Delft, The Netherlands.
His research interests include modeling, optimization, game theory, and control of complex multi-agent systems, with application in energy distribution systems, and social networks. He is author of 20+ printed international publications. He is the recipient of the 2022 IEEE CSS Italy Best Young Author Journal Paper Award.
Pubblicazioni
2024
- Scarabaggio, P., Carli, R., Grammatico, S. & Dotoli, M. (2024) Local Generalized Nash Equilibria with Nonconvex Coupling Constraints. IN IEEE Transactions on Automatic Control, .. doi:10.1109/TAC.2024.3462553
[BibTeX] [Abstract] [Download PDF]We address a class of Nash games with nonconvex coupling constraints for which we define a novel notion of local equilibrium, here named local generalized Nash equilibrium (LGNE). Our first technical contribution is to show the stability in the game theoretic sense of these equilibria on a specific local subset of the original feasible set. Remarkably, we show that the proposed notion of local equilibrium can be equivalently formulated as the solution of a quasi-variational inequality with equal Lagrange multipliers. Next, under the additional proximal smoothness assumption of the coupled feasible set, we define conditions for the existence and local uniqueness of a LGNE. To compute such an equilibrium, we propose two discrete-time dynamics, or fixed-point iterations implemented in a centralized fashion. Our third technical contribution is to prove convergence under (strongly) monotone assumptions on the pseudo- gradient mapping of the game and proximal smoothness of the coupled feasible set. Finally, we apply our theoretical results to a noncooperative version of the optimal power flow control problem. © 1963-2012 IEEE.
@ARTICLE{Scarabaggio2024, author = {Scarabaggio, Paolo and Carli, Raffaele and Grammatico, Sergio and Dotoli, Mariagrazia}, title = {Local Generalized Nash Equilibria with Nonconvex Coupling Constraints}, year = {2024}, journal = {IEEE Transactions on Automatic Control}, doi = {10.1109/TAC.2024.3462553}, url = {https://www.scopus.com/inward/record.uri?eid=2-s2.0-85204423506&doi=10.1109%2fTAC.2024.3462553&partnerID=40&md5=a06e7fdba1764046b6ce415201f6be5e}, abstract = {We address a class of Nash games with nonconvex coupling constraints for which we define a novel notion of local equilibrium, here named local generalized Nash equilibrium (LGNE). Our first technical contribution is to show the stability in the game theoretic sense of these equilibria on a specific local subset of the original feasible set. Remarkably, we show that the proposed notion of local equilibrium can be equivalently formulated as the solution of a quasi-variational inequality with equal Lagrange multipliers. Next, under the additional proximal smoothness assumption of the coupled feasible set, we define conditions for the existence and local uniqueness of a LGNE. To compute such an equilibrium, we propose two discrete-time dynamics, or fixed-point iterations implemented in a centralized fashion. Our third technical contribution is to prove convergence under (strongly) monotone assumptions on the pseudo- gradient mapping of the game and proximal smoothness of the coupled feasible set. Finally, we apply our theoretical results to a noncooperative version of the optimal power flow control problem. © 1963-2012 IEEE.}, author_keywords = {Generalized nash equilibrium; multi-agent systems; non convex generalized games; variational inequalities}, keywords = {Game theory; Coupling constraints; Feasible set; Generalized Nash equilibrium; Local equilibrium; Multiagent systems (MASs); Nash games; Non convex generalized game; Nonconvex; Technical contribution; Variational inequalities; Lagrange multipliers}, type = {Article}, publication_stage = {Article in press}, source = {Scopus}, note = {Cited by: 0; All Open Access, Hybrid Gold Open Access} }
- Zhukovskii, K., Ovsiannikova, P., Jhunjhunwala, P., Scarabaggio, P., Carli, R., Dotoli, M. & Vyatkin, V. (2024) Energy Consumption Optimisation for Horticultural Facilities IN IEEE International Conference on Emerging Technologies and Factory Automation, ETFA.. doi:10.1109/ETFA61755.2024.10710757
[BibTeX] [Abstract] [Download PDF]This paper proposes a framework designed to optimise energy consumption in vertical farming. It aims to maximise cost efficiency by balancing between minimising system operations during the electricity price peaks and the ability to trade capacity on the FCR market while also fulfilling constraints on the internal growing process. We consider that the vertical farming system has distributed control with a series of actuators controlled by various spatially distributed PLCs that we refer to as agents, to underline their independence. The paper conducts two experiments for a lO-agent system with a Pareto controller and a lOO-agent system with a Lagrangian approach and shows the balance between more cost-efficient momentary energy consumption control. © 2024 IEEE.
@CONFERENCE{Zhukovskii2024, author = {Zhukovskii, Kirill and Ovsiannikova, Polina and Jhunjhunwala, Pranay and Scarabaggio, Paolo and Carli, Raffaele and Dotoli, Mariagrazia and Vyatkin, Valeriy}, title = {Energy Consumption Optimisation for Horticultural Facilities}, year = {2024}, journal = {IEEE International Conference on Emerging Technologies and Factory Automation, ETFA}, doi = {10.1109/ETFA61755.2024.10710757}, url = {https://www.scopus.com/inward/record.uri?eid=2-s2.0-85207826602&doi=10.1109%2fETFA61755.2024.10710757&partnerID=40&md5=301035e618ab242689424422b21164c4}, abstract = {This paper proposes a framework designed to optimise energy consumption in vertical farming. It aims to maximise cost efficiency by balancing between minimising system operations during the electricity price peaks and the ability to trade capacity on the FCR market while also fulfilling constraints on the internal growing process. We consider that the vertical farming system has distributed control with a series of actuators controlled by various spatially distributed PLCs that we refer to as agents, to underline their independence. The paper conducts two experiments for a lO-agent system with a Pareto controller and a lOO-agent system with a Lagrangian approach and shows the balance between more cost-efficient momentary energy consumption control. © 2024 IEEE.}, author_keywords = {energy consumption optimisation; multi-agent systems; vertical farming}, keywords = {Agent systems; Cost-efficiency; Electricity prices; Energy consumption optimization; Energy-consumption; Farming system; Growing process; Multiagent systems (MASs); Systems operation; Vertical farming}, type = {Conference paper}, publication_stage = {Final}, source = {Scopus}, note = {Cited by: 0} }
2023
- Mignoni, N., Scarabaggio, P., Carli, R. & Dotoli, M. (2023) Control frameworks for transactive energy storage services in energy communities. IN Control Engineering Practice, 130.. doi:10.1016/j.conengprac.2022.105364
[BibTeX] [Abstract] [Download PDF]Recently, the decreasing cost of storage technologies and the emergence of economy-driven mechanisms for energy exchange are contributing to the spread of energy communities. In this context, this paper aims at defining innovative transactive control frameworks for energy communities equipped with independent service-oriented energy storage systems. The addressed control problem consists in optimally scheduling the energy activities of a group of prosumers, characterized by their own demand and renewable generation, and a group of energy storage service providers, able to store the prosumers’ energy surplus and, subsequently, release it upon a fee payment. We propose two novel resolution algorithms based on a game theoretical control formulation, a coordinated and an uncoordinated one, which can be alternatively used depending on the underlying communication architecture of the grid. The two proposed approaches are validated through numerical simulations on realistic scenarios. Results show that the use of a particular framework does not alter fairness, at least at the community level, i.e., no participant in the groups of prosumers or providers can strongly benefit from changing its strategy while compromising others’ welfare. Lastly, the approaches are compared with a centralized control method showing better computational results. © 2022 Elsevier Ltd
@ARTICLE{Mignoni2023, author = {Mignoni, Nicola and Scarabaggio, Paolo and Carli, Raffaele and Dotoli, Mariagrazia}, title = {Control frameworks for transactive energy storage services in energy communities}, year = {2023}, journal = {Control Engineering Practice}, volume = {130}, doi = {10.1016/j.conengprac.2022.105364}, url = {https://www.scopus.com/inward/record.uri?eid=2-s2.0-85140312671&doi=10.1016%2fj.conengprac.2022.105364&partnerID=40&md5=c2f86f25172d9c67e24d1f09c9b54689}, abstract = {Recently, the decreasing cost of storage technologies and the emergence of economy-driven mechanisms for energy exchange are contributing to the spread of energy communities. In this context, this paper aims at defining innovative transactive control frameworks for energy communities equipped with independent service-oriented energy storage systems. The addressed control problem consists in optimally scheduling the energy activities of a group of prosumers, characterized by their own demand and renewable generation, and a group of energy storage service providers, able to store the prosumers’ energy surplus and, subsequently, release it upon a fee payment. We propose two novel resolution algorithms based on a game theoretical control formulation, a coordinated and an uncoordinated one, which can be alternatively used depending on the underlying communication architecture of the grid. The two proposed approaches are validated through numerical simulations on realistic scenarios. Results show that the use of a particular framework does not alter fairness, at least at the community level, i.e., no participant in the groups of prosumers or providers can strongly benefit from changing its strategy while compromising others’ welfare. Lastly, the approaches are compared with a centralized control method showing better computational results. © 2022 Elsevier Ltd}, author_keywords = {Distributed control; Energy communities; Energy storage systems; Game theory; Smart grids; Transactive control; Transactive energy management}, keywords = {Computation theory; Distributed parameter control systems; Electric power transmission networks; Energy storage; Smart power grids; Control framework; Distributed-control; Energy; Energy community; Energy storage system; Smart grid; Storage services; Storage systems; Transactive controls; Transactive energy management; Game theory}, type = {Article}, publication_stage = {Final}, source = {Scopus}, note = {Cited by: 30; All Open Access, Green Open Access} }
- Proia, S., Cavone, G., Scarabaggio, P., Carli, R. & Dotoli, M. (2023) Safety Compliant, Ergonomic and Time-Optimal Trajectory Planning for Collaborative Robotics. IN IEEE Transactions on Automation Science and Engineering, .1–12. doi:10.1109/TASE.2023.3331505
[BibTeX] [Abstract] [Download PDF]The demand for safe and ergonomic workplaces is rapidly growing in modern industrial scenarios, especially for companies that intensely rely on Human-Robot Collaboration (HRC). This work focuses on optimizing the trajectory of the end-effector of a cobot arm in a collaborative industrial environment, ensuring the maximization of the operator’s safety and ergonomics without sacrificing production efficiency requirements. Hence, a multi-objective optimization strategy for trajectory planning in a safe and ergonomic HRC is defined. This approach aims at finding the best trade-off between the total traversal time of the cobot’s end-effector trajectory and ergonomics for the human worker, while respecting in the kinematic constraint of the optimization problem the ISO safety requirements through the well-known Speed and Separation Monitoring (SSM) methodology. Guaranteeing an ergonomic HRC means reducing musculoskeletal disorders linked to risky and highly repetitive activities. The three main phases of the proposed technique are described as follows. First, a manikin designed using a dedicated software is employed to evaluate the Rapid Upper Limb Assessment (RULA) ergonomic index in the working area. Next, a second-order cone programming problem is defined to represent a time-optimal safety compliant trajectory planning problem. Finally, the trajectory that ensures the best compromise between these two opposing goals –minimizing the task’s traversal time and maintaining a high level of ergonomics for the human worker– is computed by defining and solving a multi-objective control problem. The method is tested on an experimental case study in reference to an assembly task and the obtained results are discussed, showing the effectiveness of the proposed approach.
Note to Practitioners —Health and safety in workplaces are business imperatives, since they ensure not only a safe collaboration between industrial machinery and human operators, but also an increased productivity and flexibility of the entire industrial process. Hence, investing in health is a real driver for business growth. The key enabling technologies of Industry 4.0, such as collaborative robotics, exoskeletons, virtual and augmented reality, require standardization and indispensable technical safety requirements that cannot ignore physical, sensory, and psychological peculiarities of the human worker and aspects like usability and acceptability of these technologies in performing their activities. Against this ongoing industrial challenge, the aim of this paper is to provide researchers and practitioners with an innovative HRC trajectory planning methodology focused on enhancing production efficiency while respecting the SSM ISO safety requirement and guaranteeing the ergonomic optimal position of the operator during an assembly task. Therefore, the proposed methodology can be a convenient solution to be deployed in industrial companies, since it can support human operators by drastically reducing work-related musculoskeletal disorders and augmenting their performance in the working environment. Authors@ARTICLE{Proia20231, author = {Proia, Silvia and Cavone, Graziana and Scarabaggio, Paolo and Carli, Raffaele and Dotoli, Mariagrazia}, title = {Safety Compliant, Ergonomic and Time-Optimal Trajectory Planning for Collaborative Robotics}, year = {2023}, journal = {IEEE Transactions on Automation Science and Engineering}, pages = {1–12}, doi = {10.1109/TASE.2023.3331505}, url = {https://www.scopus.com/inward/record.uri?eid=2-s2.0-85178048425&doi=10.1109%2fTASE.2023.3331505&partnerID=40&md5=7fe6504b770617ce6996f3bf55c5d7c1}, abstract = {The demand for safe and ergonomic workplaces is rapidly growing in modern industrial scenarios, especially for companies that intensely rely on Human-Robot Collaboration (HRC). This work focuses on optimizing the trajectory of the end-effector of a cobot arm in a collaborative industrial environment, ensuring the maximization of the operator’s safety and ergonomics without sacrificing production efficiency requirements. Hence, a multi-objective optimization strategy for trajectory planning in a safe and ergonomic HRC is defined. This approach aims at finding the best trade-off between the total traversal time of the cobot’s end-effector trajectory and ergonomics for the human worker, while respecting in the kinematic constraint of the optimization problem the ISO safety requirements through the well-known Speed and Separation Monitoring (SSM) methodology. Guaranteeing an ergonomic HRC means reducing musculoskeletal disorders linked to risky and highly repetitive activities. The three main phases of the proposed technique are described as follows. First, a manikin designed using a dedicated software is employed to evaluate the Rapid Upper Limb Assessment (RULA) ergonomic index in the working area. Next, a second-order cone programming problem is defined to represent a time-optimal safety compliant trajectory planning problem. Finally, the trajectory that ensures the best compromise between these two opposing goals –minimizing the task’s traversal time and maintaining a high level of ergonomics for the human worker– is computed by defining and solving a multi-objective control problem. The method is tested on an experimental case study in reference to an assembly task and the obtained results are discussed, showing the effectiveness of the proposed approach.
Note to Practitioners —Health and safety in workplaces are business imperatives, since they ensure not only a safe collaboration between industrial machinery and human operators, but also an increased productivity and flexibility of the entire industrial process. Hence, investing in health is a real driver for business growth. The key enabling technologies of Industry 4.0, such as collaborative robotics, exoskeletons, virtual and augmented reality, require standardization and indispensable technical safety requirements that cannot ignore physical, sensory, and psychological peculiarities of the human worker and aspects like usability and acceptability of these technologies in performing their activities. Against this ongoing industrial challenge, the aim of this paper is to provide researchers and practitioners with an innovative HRC trajectory planning methodology focused on enhancing production efficiency while respecting the SSM ISO safety requirement and guaranteeing the ergonomic optimal position of the operator during an assembly task. Therefore, the proposed methodology can be a convenient solution to be deployed in industrial companies, since it can support human operators by drastically reducing work-related musculoskeletal disorders and augmenting their performance in the working environment. Authors}, author_keywords = {cobots; Collaboration; Collaborative robotics; Ergonomics; ergonomics; human-robot collaboration (HRC); Optimization; rapid upper limb assessment (RULA); Robots; safety; Safety; speed and separation monitoring; time-optimal trajectory planning; Trajectory; Trajectory planning}, keywords = {Accident prevention; Augmented reality; Collaborative robots; Distributed computer systems; Economic and social effects; Ergonomics; Exoskeleton (Robotics); Industrial robots; Multiobjective optimization; Occupational risks; Production efficiency; Robot programming; Virtual reality; Collaboration; Human-robot collaboration; Optimisations; Rapid upper limb assessment; Rapid upper limb assessments; Speed and separation monitoring; Time-optimal trajectory planning; Trajectory Planning; Workers'; Trajectories}, type = {Article}, publication_stage = {Article in press}, source = {Scopus}, note = {Cited by: 0; All Open Access, Hybrid Gold Open Access} } - Atrigna, M., Buonanno, A., Carli, R., Cavone, G., Scarabaggio, P., Valenti, M., Graditi, G. & Dotoli, M. (2023) A Machine Learning Approach to Fault Prediction of Power Distribution Grids Under Heatwaves. IN IEEE Transactions on Industry Applications, 59.4835 – 4845. doi:10.1109/TIA.2023.3262230
[BibTeX] [Abstract] [Download PDF]Climate change is increasing the occurrence of the so-called heatwaves with a trend that is expected to worsen in the next years due to global warming. The growing intensity and duration of these extreme weather events are leading to a significant number of power system failures, especially in urban areas. This is drastically affecting the reliability and normal operation of power distribution grids around the world, with high financial costs and huge negative impacts on people’s life. Typically, the response to these failure events is approached by post-event analysis, aimed at identifying the grid areas that require resources to increase the resilience of the system and prevent future outages. Nevertheless, understanding the nature of heatwaves and forecasting their impact on power distribution systems can be useful to anticipate them and accelerate a reaction, possibly avoiding negative impacts on power systems and customers. In this study, a structured method to predict distribution grid disruptions caused by heatwaves is defined. The proposed method relies on machine learning to analyze previous failure data and forecast power grid outages using operational and meteorological information. The method is evaluated using real failure data from a large power distribution network located in southern Italy. © 1972-2012 IEEE.
@ARTICLE{Atrigna20234835, author = {Atrigna, Mauro and Buonanno, Amedeo and Carli, Raffaele and Cavone, Graziana and Scarabaggio, Paolo and Valenti, Maria and Graditi, Giorgio and Dotoli, Mariagrazia}, title = {A Machine Learning Approach to Fault Prediction of Power Distribution Grids Under Heatwaves}, year = {2023}, journal = {IEEE Transactions on Industry Applications}, volume = {59}, number = {4}, pages = {4835 – 4845}, doi = {10.1109/TIA.2023.3262230}, url = {https://www.scopus.com/inward/record.uri?eid=2-s2.0-85151511272&doi=10.1109%2fTIA.2023.3262230&partnerID=40&md5=89991feff02c7dffe5f98c9f90e0be87}, abstract = {Climate change is increasing the occurrence of the so-called heatwaves with a trend that is expected to worsen in the next years due to global warming. The growing intensity and duration of these extreme weather events are leading to a significant number of power system failures, especially in urban areas. This is drastically affecting the reliability and normal operation of power distribution grids around the world, with high financial costs and huge negative impacts on people's life. Typically, the response to these failure events is approached by post-event analysis, aimed at identifying the grid areas that require resources to increase the resilience of the system and prevent future outages. Nevertheless, understanding the nature of heatwaves and forecasting their impact on power distribution systems can be useful to anticipate them and accelerate a reaction, possibly avoiding negative impacts on power systems and customers. In this study, a structured method to predict distribution grid disruptions caused by heatwaves is defined. The proposed method relies on machine learning to analyze previous failure data and forecast power grid outages using operational and meteorological information. The method is evaluated using real failure data from a large power distribution network located in southern Italy. © 1972-2012 IEEE.}, author_keywords = {condition monitoring; fault prediction; heatwaves; machine learning; power system failures; Power system reliability}, keywords = {Artificial intelligence; Forecasting; Global warming; Learning systems; Outages; Fault prediction; Heating system; Heatwaves; Machine-learning; Power; Power cables; Power system; Power system failures; Power systems reliability; Resilience; Condition monitoring}, type = {Article}, publication_stage = {Final}, source = {Scopus}, note = {Cited by: 13} }
- Cavone, G., Stella, S., Scarabaggio, P., Carli, R., Lisi, S., Garavelli, A. C. & Dotoli, M. (2023) A Colored Petri Net Tool for the Design of Robotic Palletizing Cells IN 9th 2023 International Conference on Control, Decision and Information Technologies, CoDIT 2023., 12 – 17. doi:10.1109/CoDIT58514.2023.10284186
[BibTeX] [Abstract] [Download PDF]Driven by the digital transformation required by Logistics 4.0, the use of automation in warehouses is constantly growing. In particular, robotic palletizers offer significant potential for optimizing warehouse operations, thanks to higher flexibility and throughput than traditional palletizing systems. Despite the availability of several solutions in the market, the optimal deployment of a robotic palletizer in warehouses is not straightforward: a design phase is needed to determine the most convenient configuration that ensures automatic palletizing is fully integrated into the warehouse processes. In this paper, we propose a simulation-based versatile tool for modeling and analysis purposes, aimed at supporting the design of a robotic palletizing cell in a bottom-up fashion. As a core methodology, we employ timed colored Petri nets, which allow – once the analysis on packing requirements and constraints is conducted – to rapidly model the system as a composition of basic subsystems, and implement alternative simulations to evaluate the corresponding performance and effectively benchmark the alternative configurations. The proposed approach is applied to a real case study, showing its effectiveness in identifying the solution that achieves a good compromise between the use of resources and the performance of warehouse operations. © 2023 IEEE.
@CONFERENCE{Cavone202312, author = {Cavone, Graziana and Stella, Silvia and Scarabaggio, Paolo and Carli, Raffaele and Lisi, Stefano and Garavelli, Achille Claudio and Dotoli, Mariagrazia}, title = {A Colored Petri Net Tool for the Design of Robotic Palletizing Cells}, year = {2023}, journal = {9th 2023 International Conference on Control, Decision and Information Technologies, CoDIT 2023}, pages = {12 – 17}, doi = {10.1109/CoDIT58514.2023.10284186}, url = {https://www.scopus.com/inward/record.uri?eid=2-s2.0-85177446511&doi=10.1109%2fCoDIT58514.2023.10284186&partnerID=40&md5=fb1f951ba12c45828607bf01cf994fff}, abstract = {Driven by the digital transformation required by Logistics 4.0, the use of automation in warehouses is constantly growing. In particular, robotic palletizers offer significant potential for optimizing warehouse operations, thanks to higher flexibility and throughput than traditional palletizing systems. Despite the availability of several solutions in the market, the optimal deployment of a robotic palletizer in warehouses is not straightforward: a design phase is needed to determine the most convenient configuration that ensures automatic palletizing is fully integrated into the warehouse processes. In this paper, we propose a simulation-based versatile tool for modeling and analysis purposes, aimed at supporting the design of a robotic palletizing cell in a bottom-up fashion. As a core methodology, we employ timed colored Petri nets, which allow - once the analysis on packing requirements and constraints is conducted - to rapidly model the system as a composition of basic subsystems, and implement alternative simulations to evaluate the corresponding performance and effectively benchmark the alternative configurations. The proposed approach is applied to a real case study, showing its effectiveness in identifying the solution that achieves a good compromise between the use of resources and the performance of warehouse operations. © 2023 IEEE.}, author_keywords = {Colored Petri net; Discrete Event Systems; Modeling and Simulation; Robotic Palletizing System}, keywords = {Benchmarking; Petri nets; Robotics; Warehouses; Colored Petri Nets; Digital transformation; Discrete events systems; High-throughput; Model and simulation; Performance; Robotic palletizers; Robotic palletizing system; Robotic-palletizing cell; Warehouse operation; Discrete event simulation}, type = {Conference paper}, publication_stage = {Final}, source = {Scopus}, note = {Cited by: 0} }
2022
- Scarabaggio, P., Carli, R., Parisio, A. & Dotoli, M. (2022) On Controlling Battery Degradation in Vehicle-to-Grid Energy Markets IN IEEE International Conference on Automation Science and Engineering., 1206 – 1211. doi:10.1109/CASE49997.2022.9926729
[BibTeX] [Abstract] [Download PDF]Nowadays, power grids are facing reduced total system inertia as traditional generators are phased out in favor of renewable energy sources. This issue is expected to deepen with the increasing penetration of electric vehicles (EVs). The influence of a single EV on power networks is low; nevertheless, the aggregate impact becomes relevant when they are properly coordinated. In this context, we consider the frequent case of a group of EVs connected to a parking lot with a photovoltaic facility. We propose a novel strategy to optimally control their batteries during the parking session, which is able to satisfy their requirements and energy constraints. EVs participate in a noncooperative energy market based on a smart pricing mechanism that is designed in order to increase the predictability and flexibility of the aggregate parking load. Differently from the existing contributions, we employ a novel approach to minimize the degradation of batteries. The effectiveness of the proposed method is validated through numerical experiments based on a real scenario. © 2022 IEEE.
@CONFERENCE{Scarabaggio20221206, author = {Scarabaggio, Paolo and Carli, Raffaele and Parisio, Alessandra and Dotoli, Mariagrazia}, title = {On Controlling Battery Degradation in Vehicle-to-Grid Energy Markets}, year = {2022}, journal = {IEEE International Conference on Automation Science and Engineering}, volume = {2022-August}, pages = {1206 – 1211}, doi = {10.1109/CASE49997.2022.9926729}, url = {https://www.scopus.com/inward/record.uri?eid=2-s2.0-85141672066&doi=10.1109%2fCASE49997.2022.9926729&partnerID=40&md5=ed24721222037df90795b0e4d7a1cbb1}, abstract = {Nowadays, power grids are facing reduced total system inertia as traditional generators are phased out in favor of renewable energy sources. This issue is expected to deepen with the increasing penetration of electric vehicles (EVs). The influence of a single EV on power networks is low; nevertheless, the aggregate impact becomes relevant when they are properly coordinated. In this context, we consider the frequent case of a group of EVs connected to a parking lot with a photovoltaic facility. We propose a novel strategy to optimally control their batteries during the parking session, which is able to satisfy their requirements and energy constraints. EVs participate in a noncooperative energy market based on a smart pricing mechanism that is designed in order to increase the predictability and flexibility of the aggregate parking load. Differently from the existing contributions, we employ a novel approach to minimize the degradation of batteries. The effectiveness of the proposed method is validated through numerical experiments based on a real scenario. © 2022 IEEE.}, author_keywords = {charging scheduling; Electric vehicles; model predictive control}, keywords = {Aggregates; Charging (batteries); Electric power transmission networks; Electric vehicles; Numerical methods; Renewable energy resources; Secondary batteries; Vehicle-to-grid; Battery degradation; Charging scheduling; Energy markets; Model-predictive control; Parking lots; Power grids; Power networks; Renewable energy source; System inertia; Vehicle to grids; Model predictive control}, type = {Conference paper}, publication_stage = {Final}, source = {Scopus}, note = {Cited by: 0} }
- Scarabaggio, P., Carli, R., Cavone, G., Epicoco, N. & Dotoli, M. (2022) Nonpharmaceutical Stochastic Optimal Control Strategies to Mitigate the COVID-19 Spread. IN IEEE Transactions on Automation Science and Engineering, 19.560 – 575. doi:10.1109/TASE.2021.3111338
[BibTeX] [Abstract] [Download PDF]This article proposes a stochastic nonlinear model predictive controller to support policymakers in determining robust optimal nonpharmaceutical strategies to tackle the COVID-19 pandemic waves. First, a time-varying SIRCQTHE epidemiological model is defined to get predictions on the pandemic dynamics. A stochastic model predictive control problem is then formulated to select the necessary control actions (i.e., restrictions on the mobility for different socioeconomic categories) to minimize the socioeconomic costs. In particular, considering the uncertainty characterizing this decision-making process, we ensure that the capacity of the healthcare system is not violated in accordance with a chance constraint approach. The effectiveness of the presented method in properly supporting the definition of diversified nonpharmaceutical strategies for tackling the COVID-19 spread is tested on the network of Italian regions using real data. The proposed approach can be easily extended to cope with other countries’ characteristics and different levels of the spatial scale. Note to Practitioners – This article is motivated by the emerging need for developing effective methods to support policymakers in mitigating the effects of the COVID-19 pandemic. The proposed feedback control strategy – combining a multiregion epidemiological model with a nonlinear stochastic model predictive control approach – allows the robust identification of the most effective restrictive measures considering the corresponding effects on the healthcare and socioeconomic systems. The proposed framework is a general and flexible method that can be applied to various real scenarios, leveraging mobility data, available from the Google mobility service, to recognize patterns and predict future behaviors of individuals. © 2004-2012 IEEE.
@ARTICLE{Scarabaggio2022560, author = {Scarabaggio, Paolo and Carli, Raffaele and Cavone, Graziana and Epicoco, Nicola and Dotoli, Mariagrazia}, title = {Nonpharmaceutical Stochastic Optimal Control Strategies to Mitigate the COVID-19 Spread}, year = {2022}, journal = {IEEE Transactions on Automation Science and Engineering}, volume = {19}, number = {2}, pages = {560 – 575}, doi = {10.1109/TASE.2021.3111338}, url = {https://www.scopus.com/inward/record.uri?eid=2-s2.0-85115686920&doi=10.1109%2fTASE.2021.3111338&partnerID=40&md5=ef02a3b0f8f2eeff0ec2f0e0a861a0b8}, abstract = {This article proposes a stochastic nonlinear model predictive controller to support policymakers in determining robust optimal nonpharmaceutical strategies to tackle the COVID-19 pandemic waves. First, a time-varying SIRCQTHE epidemiological model is defined to get predictions on the pandemic dynamics. A stochastic model predictive control problem is then formulated to select the necessary control actions (i.e., restrictions on the mobility for different socioeconomic categories) to minimize the socioeconomic costs. In particular, considering the uncertainty characterizing this decision-making process, we ensure that the capacity of the healthcare system is not violated in accordance with a chance constraint approach. The effectiveness of the presented method in properly supporting the definition of diversified nonpharmaceutical strategies for tackling the COVID-19 spread is tested on the network of Italian regions using real data. The proposed approach can be easily extended to cope with other countries' characteristics and different levels of the spatial scale. Note to Practitioners - This article is motivated by the emerging need for developing effective methods to support policymakers in mitigating the effects of the COVID-19 pandemic. The proposed feedback control strategy - combining a multiregion epidemiological model with a nonlinear stochastic model predictive control approach - allows the robust identification of the most effective restrictive measures considering the corresponding effects on the healthcare and socioeconomic systems. The proposed framework is a general and flexible method that can be applied to various real scenarios, leveraging mobility data, available from the Google mobility service, to recognize patterns and predict future behaviors of individuals. © 2004-2012 IEEE.}, author_keywords = {COVID-19; epidemic control; mitigation strategies; pandemic modeling; stochastic model predictive control (MPC)}, keywords = {Decision making; Disease control; Model predictive control; Optimization; Predictive control systems; Random processes; Stochastic control systems; Stochastic models; COVID-19; Epidemic control; Medical services; Mitigation strategy; Pandemic; Pandemic modeling; Predictive models; Stochastic model predictive control .; Stochastic model predictive controls; Uncertainty; Stochastic systems}, type = {Article}, publication_stage = {Final}, source = {Scopus}, note = {Cited by: 22; All Open Access, Green Open Access} }
- Scarabaggio, P., Grammatico, S., Carli, R. & Dotoli, M. (2022) Distributed Demand Side Management with Stochastic Wind Power Forecasting. IN IEEE Transactions on Control Systems Technology, 30.97 – 112. doi:10.1109/TCST.2021.3056751
[BibTeX] [Abstract] [Download PDF]In this article, we propose a distributed demand-side management (DSM) approach for smart grids taking into account uncertainty in wind power forecasting. The smart grid model comprehends traditional users as well as active users (prosumers). Through a rolling-horizon approach, prosumers participate in a DSM program, aiming at minimizing their cost in the presence of uncertain wind power generation by a game theory approach. We assume that each user selfishly formulates its grid optimization problem as a noncooperative game. The core challenge in this article is defining an approach to cope with the uncertainty in wind power availability. We tackle this issue from two different sides: by employing the expected value to define a deterministic counterpart for the problem and by adopting a stochastic approximated framework. In the latter case, we employ the sample average approximation (SAA) technique, whose results are based on a probability density function (PDF) for the wind speed forecasts. We improve the PDF by using historical wind speed data, and by employing a control index that takes into account the weather condition stability. Numerical simulations on a real data set show that the proposed stochastic strategy generates lower individual costs compared to the standard expected value approach. © 1993-2012 IEEE.
@ARTICLE{Scarabaggio202297, author = {Scarabaggio, Paolo and Grammatico, Sergio and Carli, Raffaele and Dotoli, Mariagrazia}, title = {Distributed Demand Side Management with Stochastic Wind Power Forecasting}, year = {2022}, journal = {IEEE Transactions on Control Systems Technology}, volume = {30}, number = {1}, pages = {97 – 112}, doi = {10.1109/TCST.2021.3056751}, url = {https://www.scopus.com/inward/record.uri?eid=2-s2.0-85100918524&doi=10.1109%2fTCST.2021.3056751&partnerID=40&md5=4f491e205e325ad467291e71ce8dbff8}, abstract = {In this article, we propose a distributed demand-side management (DSM) approach for smart grids taking into account uncertainty in wind power forecasting. The smart grid model comprehends traditional users as well as active users (prosumers). Through a rolling-horizon approach, prosumers participate in a DSM program, aiming at minimizing their cost in the presence of uncertain wind power generation by a game theory approach. We assume that each user selfishly formulates its grid optimization problem as a noncooperative game. The core challenge in this article is defining an approach to cope with the uncertainty in wind power availability. We tackle this issue from two different sides: by employing the expected value to define a deterministic counterpart for the problem and by adopting a stochastic approximated framework. In the latter case, we employ the sample average approximation (SAA) technique, whose results are based on a probability density function (PDF) for the wind speed forecasts. We improve the PDF by using historical wind speed data, and by employing a control index that takes into account the weather condition stability. Numerical simulations on a real data set show that the proposed stochastic strategy generates lower individual costs compared to the standard expected value approach. © 1993-2012 IEEE.}, author_keywords = {Demand-side management (DSM); model predictive control; sample average approximation (SAA); smart grid; stochastic optimization}, keywords = {Electric power generation; Electric power transmission networks; Electric utilities; Game theory; Probability density function; Smart power grids; Stochastic systems; Weather forecasting; Wind; Wind power; Grid optimization; Noncooperative game; Probability density function (pdf); Sample average approximation; Stochastic winds; Wind power availability; Wind power forecasting; Wind speed forecast; Demand side management}, type = {Article}, publication_stage = {Final}, source = {Scopus}, note = {Cited by: 88; All Open Access, Green Open Access} }
- Scarabaggio, P., Carli, R. & Dotoli, M. (2022) Noncooperative Equilibrium-Seeking in Distributed Energy Systems under AC Power Flow Nonlinear Constraints. IN IEEE Transactions on Control of Network Systems, 9.1731 – 1742. doi:10.1109/TCNS.2022.3181527
[BibTeX] [Abstract] [Download PDF]Power distribution grids are commonly controlled through centralized approaches, such as the optimal power flow. However, the current pervasive deployment of distributed renewable energy sources and the increasing growth of active players, providing ancillary services to the grid, have made these centralized frameworks no longer appropriate. In this context, we propose a novel noncooperative control mechanism for optimally regulating the operation of power distribution networks equipped with traditional loads, distributed generation, and active users. The latter, also known as prosumers, contribute to the grid optimization process by leveraging their flexible demand, dispatchable generation capability, and/or energy storage potential. Active users participate in a noncooperative liberalized market designed to increase the penetration of renewable generation and improve the predictability of power injection from the high-voltage grid. The novelty of our game-theoretical approach consists in incorporating economic factors as well as physical constraints and grid stability aspects. Finally, by integrating the proposed framework into a rolling-horizon approach, we show its effectiveness and resiliency through numerical experiments. © 2014 IEEE.
@ARTICLE{Scarabaggio20221731, author = {Scarabaggio, Paolo and Carli, Raffaele and Dotoli, Mariagrazia}, title = {Noncooperative Equilibrium-Seeking in Distributed Energy Systems under AC Power Flow Nonlinear Constraints}, year = {2022}, journal = {IEEE Transactions on Control of Network Systems}, volume = {9}, number = {4}, pages = {1731 – 1742}, doi = {10.1109/TCNS.2022.3181527}, url = {https://www.scopus.com/inward/record.uri?eid=2-s2.0-85132727510&doi=10.1109%2fTCNS.2022.3181527&partnerID=40&md5=01b1c79aa91772c7e9920d019e70b5b8}, abstract = {Power distribution grids are commonly controlled through centralized approaches, such as the optimal power flow. However, the current pervasive deployment of distributed renewable energy sources and the increasing growth of active players, providing ancillary services to the grid, have made these centralized frameworks no longer appropriate. In this context, we propose a novel noncooperative control mechanism for optimally regulating the operation of power distribution networks equipped with traditional loads, distributed generation, and active users. The latter, also known as prosumers, contribute to the grid optimization process by leveraging their flexible demand, dispatchable generation capability, and/or energy storage potential. Active users participate in a noncooperative liberalized market designed to increase the penetration of renewable generation and improve the predictability of power injection from the high-voltage grid. The novelty of our game-theoretical approach consists in incorporating economic factors as well as physical constraints and grid stability aspects. Finally, by integrating the proposed framework into a rolling-horizon approach, we show its effectiveness and resiliency through numerical experiments. © 2014 IEEE.}, author_keywords = {AC power flow; distributed control; electric power networks; game theory}, keywords = {Distributed parameter control systems; Distributed power generation; Electric load flow; Electric load management; Electric network analysis; Electric power system control; Electric power transmission networks; Natural resources; Renewable energy resources; AC power flow; Distributed energy systems; Distributed-control; Electric power networks; Generator; Load modeling; Network systems; Non-linear constraints; Power grids; Renewable energy source; Game theory}, type = {Article}, publication_stage = {Final}, source = {Scopus}, note = {Cited by: 34; All Open Access, Green Open Access, Hybrid Gold Open Access} }
- Mignoni, N., Scarabaggio, P., Carli, R. & Dotoli, M. (2022) Game Theoretical Control Frameworks for Multiple Energy Storage Services in Energy Communities IN 2022 8th International Conference on Control, Decision and Information Technologies, CoDIT 2022., 1580 – 1585. doi:10.1109/CoDIT55151.2022.9804087
[BibTeX] [Abstract] [Download PDF]In the last decade, distributed energy generation and storage have significantly contributed to the widespread of energy communities. In this context, we propose an energy community model constituted by prosumers, characterized by their own demand and renewable generation, and service-oriented energy storage providers, able to store energy surplus and release it upon a fee payment. We address the problem of optimally schedule the energy flows in the community, with the final goal of making the prosumers’ energy supply more efficient, while creating a sustainable and profitable business model for storage providers. The proposed resolution algorithms are based on decentralized and distributed game theoretical control schemes. These approaches are mathematically formulated and then effectively validated and compared with a centralized method through numerical simulations on realistic scenarios. © 2022 IEEE.
@CONFERENCE{Mignoni20221580, author = {Mignoni, Nicola and Scarabaggio, Paolo and Carli, Raffaele and Dotoli, Mariagrazia}, title = {Game Theoretical Control Frameworks for Multiple Energy Storage Services in Energy Communities}, year = {2022}, journal = {2022 8th International Conference on Control, Decision and Information Technologies, CoDIT 2022}, pages = {1580 – 1585}, doi = {10.1109/CoDIT55151.2022.9804087}, url = {https://www.scopus.com/inward/record.uri?eid=2-s2.0-85134303591&doi=10.1109%2fCoDIT55151.2022.9804087&partnerID=40&md5=c5c686570c9998d9639b765af7b24245}, abstract = {In the last decade, distributed energy generation and storage have significantly contributed to the widespread of energy communities. In this context, we propose an energy community model constituted by prosumers, characterized by their own demand and renewable generation, and service-oriented energy storage providers, able to store energy surplus and release it upon a fee payment. We address the problem of optimally schedule the energy flows in the community, with the final goal of making the prosumers' energy supply more efficient, while creating a sustainable and profitable business model for storage providers. The proposed resolution algorithms are based on decentralized and distributed game theoretical control schemes. These approaches are mathematically formulated and then effectively validated and compared with a centralized method through numerical simulations on realistic scenarios. © 2022 IEEE.}, keywords = {Game theory; Numerical methods; Community model; Control framework; Demand generation; Distributed energy generation and storages; Energy; Energy flow; Energy supplies; Renewable generation; Service Oriented; Storage services; Energy storage}, type = {Conference paper}, publication_stage = {Final}, source = {Scopus}, note = {Cited by: 1} }
- Nasiri, F., Ooka, R., Haghighat, F., Shirzadi, N., Dotoli, M., Carli, R., Scarabaggio, P., Behzadi, A., Rahnama, S., Afshari, A., Kuznik, F., Fabrizio, E., Choudhary, R. & Sadrizadeh, S. (2022) Data Analytics and Information Technologies for Smart Energy Storage Systems: A State-of-the-Art Review. IN Sustainable Cities and Society, 84.. doi:10.1016/j.scs.2022.104004
[BibTeX] [Abstract] [Download PDF]This article provides a state-of-the-art review on emerging applications of smart tools such as data analytics and smart technologies such as internet-of-things in case of design, management and control of energy storage systems. In particular, we have established a classification of the types and targets of various predictive analytics for estimation of load, energy prices, renewable energy inputs, state of the charge, fault diagnosis, etc. In addition, the applications of information technologies, and in particular, use of cloud, internet-of-things, building management systems and building information modeling and their contributions to management of energy storage systems will be reviewed in details. The paper concludes by highlighting the emerging issues in smart energy storage systems and providing directions for future research. © 2022 Elsevier Ltd
@ARTICLE{Nasiri2022, author = {Nasiri, Fuzhan and Ooka, Ryozo and Haghighat, Fariborz and Shirzadi, Navid and Dotoli, Mariagrazia and Carli, Raffaele and Scarabaggio, Paolo and Behzadi, Amirmohammad and Rahnama, Samira and Afshari, Alireza and Kuznik, Frédéric and Fabrizio, Enrico and Choudhary, Ruchi and Sadrizadeh, Sasan}, title = {Data Analytics and Information Technologies for Smart Energy Storage Systems: A State-of-the-Art Review}, year = {2022}, journal = {Sustainable Cities and Society}, volume = {84}, doi = {10.1016/j.scs.2022.104004}, url = {https://www.scopus.com/inward/record.uri?eid=2-s2.0-85133235991&doi=10.1016%2fj.scs.2022.104004&partnerID=40&md5=c1409a9f5cd360d29ca6e0f1bf7f9fe9}, abstract = {This article provides a state-of-the-art review on emerging applications of smart tools such as data analytics and smart technologies such as internet-of-things in case of design, management and control of energy storage systems. In particular, we have established a classification of the types and targets of various predictive analytics for estimation of load, energy prices, renewable energy inputs, state of the charge, fault diagnosis, etc. In addition, the applications of information technologies, and in particular, use of cloud, internet-of-things, building management systems and building information modeling and their contributions to management of energy storage systems will be reviewed in details. The paper concludes by highlighting the emerging issues in smart energy storage systems and providing directions for future research. © 2022 Elsevier Ltd}, author_keywords = {Artificial Intelligence; Data Analytics; Energy Storage; Information Technology; Renewable Energy Intermittency; Smart Systems}, keywords = {Classification (of information); Digital storage; Energy storage; Information management; Internet of things; Predictive analytics; Data analytics; Data informations; Emerging applications; Intermittency; Renewable energies; Renewable energy intermittency; Smart energies; Smart System; State-of-the art reviews; Storage systems; alternative energy; artificial intelligence; data processing; design; energy storage; information technology; Data Analytics}, type = {Article}, publication_stage = {Final}, source = {Scopus}, note = {Cited by: 12} }
2021
- Atrigna, M., Buonanno, A., Carli, R., Cavone, G., Scarabaggio, P., Valenti, M., Graditi, G. & Dotoli, M. (2021) Effects of Heatwaves on the Failure of Power Distribution Grids: A Fault Prediction System Based on Machine Learning IN 21st IEEE International Conference on Environment and Electrical Engineering and 2021 5th IEEE Industrial and Commercial Power System Europe, EEEIC / I and CPS Europe 2021 – Proceedings.. doi:10.1109/EEEIC/ICPSEurope51590.2021.9584751
[BibTeX] [Abstract] [Download PDF]Nowadays, a power system failure can drastically affect the reliability and normal operation of power distribution grids. The preparation for these failure events is currently approached with post-event analysis to identify the area of the system that requires the most resources in order to prevent future failures. Nevertheless, the forecasting of such events can be useful to anticipate the failure and possibly avoid it. In this work, we employ several machine learning approaches to analyze historical failure data and predict power grid outages based on operational and meteorological data. The approach is tested with real failure data of a power distribution network in the South of Italy, demonstrating advantageous results also to determine areas requiring particular attention. © 2021 IEEE
@CONFERENCE{Atrigna2021, author = {Atrigna, Mauro and Buonanno, Amedeo and Carli, Raffaele and Cavone, Graziana and Scarabaggio, Paolo and Valenti, Maria and Graditi, Giorgio and Dotoli, Mariagrazia}, title = {Effects of Heatwaves on the Failure of Power Distribution Grids: A Fault Prediction System Based on Machine Learning}, year = {2021}, journal = {21st IEEE International Conference on Environment and Electrical Engineering and 2021 5th IEEE Industrial and Commercial Power System Europe, EEEIC / I and CPS Europe 2021 - Proceedings}, doi = {10.1109/EEEIC/ICPSEurope51590.2021.9584751}, url = {https://www.scopus.com/inward/record.uri?eid=2-s2.0-85126456706&doi=10.1109%2fEEEIC%2fICPSEurope51590.2021.9584751&partnerID=40&md5=fc9788f00e259fdaabf0cb50c06253be}, abstract = {Nowadays, a power system failure can drastically affect the reliability and normal operation of power distribution grids. The preparation for these failure events is currently approached with post-event analysis to identify the area of the system that requires the most resources in order to prevent future failures. Nevertheless, the forecasting of such events can be useful to anticipate the failure and possibly avoid it. In this work, we employ several machine learning approaches to analyze historical failure data and predict power grid outages based on operational and meteorological data. The approach is tested with real failure data of a power distribution network in the South of Italy, demonstrating advantageous results also to determine areas requiring particular attention. © 2021 IEEE}, author_keywords = {machine learning; Power system failures; Power system reliability}, keywords = {Electric power transmission networks; Failure (mechanical); Forecasting; Machine learning; Meteorology; Systems engineering; Failure data; Fault prediction; Heatwaves; Machine-learning; Normal operations; On-machines; Power distribution grids; Power system failures; Power systems reliability; Prediction systems; Outages}, type = {Conference paper}, publication_stage = {Final}, source = {Scopus}, note = {Cited by: 4} }
- Calefati, M., Proia, S., Scarabaggio, P., Carli, R. & Dotoli, M. (2021) A Decentralized Noncooperative Control Approach for Sharing Energy Storage Systems in Energy Communities IN Conference Proceedings – IEEE International Conference on Systems, Man and Cybernetics., 1430 – 1435. doi:10.1109/SMC52423.2021.9658851
[BibTeX] [Abstract] [Download PDF]This paper focuses on the optimal scheduling of the charging and discharging strategies of a community energy storage (CES) system, which is shared by the prosumers belonging to a grid-connected energy community. The prosumers own renewable energy sources (RESs), while they can buy/sell their energy imbalance directly from/to the power grid. For the sake of increasing the penetration of RESs and reducing the operating cost, prosumers leverage on the shared CES: in particular, each user can only employ a portion of the overall CES charge/discharge profile. Differently from the related literature, where storage devices are individually owned and the battery degradation aspects are typically disregarded, we propose a novel control mechanism based on noncooperative game theory, which allows users to minimize their energy cost as well as concur on the CES resources allocation with minimal-degradation. The effectiveness of the method is validated through numerical experiments on a realistic case study, where a shared CES supplies energy to the local community of residential prosumers. Finally, the comparison with a centralized control approach shows that the proposed framework allows all prosumers to achieve a fair cost-optimal utilization of the shared CES. © 2021 IEEE.
@CONFERENCE{Calefati20211430, author = {Calefati, Marino and Proia, Silvia and Scarabaggio, Paolo and Carli, Raffaele and Dotoli, Mariagrazia}, title = {A Decentralized Noncooperative Control Approach for Sharing Energy Storage Systems in Energy Communities}, year = {2021}, journal = {Conference Proceedings - IEEE International Conference on Systems, Man and Cybernetics}, pages = {1430 – 1435}, doi = {10.1109/SMC52423.2021.9658851}, url = {https://www.scopus.com/inward/record.uri?eid=2-s2.0-85124302216&doi=10.1109%2fSMC52423.2021.9658851&partnerID=40&md5=9472abc4fceb04dd351c66a9d3b2a30b}, abstract = {This paper focuses on the optimal scheduling of the charging and discharging strategies of a community energy storage (CES) system, which is shared by the prosumers belonging to a grid-connected energy community. The prosumers own renewable energy sources (RESs), while they can buy/sell their energy imbalance directly from/to the power grid. For the sake of increasing the penetration of RESs and reducing the operating cost, prosumers leverage on the shared CES: in particular, each user can only employ a portion of the overall CES charge/discharge profile. Differently from the related literature, where storage devices are individually owned and the battery degradation aspects are typically disregarded, we propose a novel control mechanism based on noncooperative game theory, which allows users to minimize their energy cost as well as concur on the CES resources allocation with minimal-degradation. The effectiveness of the method is validated through numerical experiments on a realistic case study, where a shared CES supplies energy to the local community of residential prosumers. Finally, the comparison with a centralized control approach shows that the proposed framework allows all prosumers to achieve a fair cost-optimal utilization of the shared CES. © 2021 IEEE.}, author_keywords = {decentralized control; energy community; energy storage; game theory; noncooperative control}, keywords = {Decentralized control; Electric power transmission networks; Energy storage; Numerical methods; Renewable energy resources; Smart power grids; Virtual storage; Community energy; Control approach; Decentralised; Decentralised control; Energy; Energy community; Noncooperative control; Optimal scheduling; Renewable energy source; Storage systems; Game theory}, type = {Conference paper}, publication_stage = {Final}, source = {Scopus}, note = {Cited by: 1} }
- Scarabaggio, P., Carli, R., Jantzen, J. & Dotoli, M. (2021) Stochastic model predictive control of community energy storage under high renewable penetration IN 2021 29th Mediterranean Conference on Control and Automation, MED 2021., 973 – 978. doi:10.1109/MED51440.2021.9480353
[BibTeX] [Abstract] [Download PDF]This paper focuses on the robust optimal on-line scheduling of a grid-connected energy community, where users are equipped with non-controllable (NCLs) and controllable loads (CLs) and share renewable energy sources (RESs) and a community energy storage system (CESS). Leveraging on the pricing signals gathered from the power grid and the predicted values for local production and demand, the energy activities inside the community are decided by a community energy manager. Differently from literature contributions commonly focused on deterministic optimal control schemes, to cope with the uncertainty that affects the forecast of the inflexible demand profile and the renewable production curve, we propose a Stochastic Model Predictive Control (MPC) approach aimed at minimizing the community energy costs. The effectiveness of the method is validated through numerical experiments on the marina of Ballen, Samso (Denmark). The comparison with a standard deterministic optimal control approach shows that the proposed stochastic MPC achieves higher performance in terms of minimized energy cost and maximized self-consumption of on-site production. © 2021 IEEE.
@CONFERENCE{Scarabaggio2021973, author = {Scarabaggio, Paolo and Carli, Raffaele and Jantzen, Jan and Dotoli, Mariagrazia}, title = {Stochastic model predictive control of community energy storage under high renewable penetration}, year = {2021}, journal = {2021 29th Mediterranean Conference on Control and Automation, MED 2021}, pages = {973 – 978}, doi = {10.1109/MED51440.2021.9480353}, url = {https://www.scopus.com/inward/record.uri?eid=2-s2.0-85113644274&doi=10.1109%2fMED51440.2021.9480353&partnerID=40&md5=6156faadbaf382124749771a715a60df}, abstract = {This paper focuses on the robust optimal on-line scheduling of a grid-connected energy community, where users are equipped with non-controllable (NCLs) and controllable loads (CLs) and share renewable energy sources (RESs) and a community energy storage system (CESS). Leveraging on the pricing signals gathered from the power grid and the predicted values for local production and demand, the energy activities inside the community are decided by a community energy manager. Differently from literature contributions commonly focused on deterministic optimal control schemes, to cope with the uncertainty that affects the forecast of the inflexible demand profile and the renewable production curve, we propose a Stochastic Model Predictive Control (MPC) approach aimed at minimizing the community energy costs. The effectiveness of the method is validated through numerical experiments on the marina of Ballen, Samso (Denmark). The comparison with a standard deterministic optimal control approach shows that the proposed stochastic MPC achieves higher performance in terms of minimized energy cost and maximized self-consumption of on-site production. © 2021 IEEE.}, author_keywords = {Community energy storage; Community renewables; Energy community; Energy management; On-line energy scheduling; Stochastic model predictive control}, keywords = {Electric power transmission networks; Energy storage; Model predictive control; Numerical methods; Optimization; Predictive control systems; Renewable energy resources; Stochastic control systems; Stochastic systems; Comparison with a standard; Controllable loads; Local production; Numerical experiments; On-site production; Online scheduling; Optimal control scheme; Renewable energy source; Stochastic models}, type = {Conference paper}, publication_stage = {Final}, source = {Scopus}, note = {Cited by: 11} }
- Scarabaggio, P., Carli, R., Cavone, G., Epicoco, N. & Dotoli, M. (2021) Modeling, estimation, and analysis of COVID-19 secondary waves: The Case of the Italian Country IN 2021 29th Mediterranean Conference on Control and Automation, MED 2021., 794 – 800. doi:10.1109/MED51440.2021.9480319
[BibTeX] [Abstract] [Download PDF]The recent trends of the COVID-19 research have been devoted to disease transmission modeling, with the aim of investigating the effects of different mitigation strategies mainly through scenario-based simulations. In this context we propose a novel non-linear time-varying model that effectively supports policy-makers in predicting and analyzing the dynamics of COVID-19 secondary waves. Specifically, this paper proposes an accurate SIRUCQTHE epidemiological model to get reliable predictions on the pandemic dynamics. Differently from the related literature, in the fitting phase, we make use of the google mobility reports to identify and predict the evolution of the infection rate. The effectiveness of the presented method is tested on the network of Italian regions. First, we describe the Italian epidemiological scenario in the COVID-19 second wave of contagions, showing the raw data available for the Italian scenario and discussing the main assumptions on the system parameters. Then, we present the different steps of the procedure used for the dynamical fitting of the SIRUCQTHE model. Finally, we compare the estimation results with the real data on the COVID-19 secondary waves in Italy. Provided the availability of reliable data to calibrate the model in heterogeneous scenarios, the proposed approach can be easily extended to cope with other scenarios. © 2021 IEEE.
@CONFERENCE{Scarabaggio2021794, author = {Scarabaggio, Paolo and Carli, Raffaele and Cavone, Graziana and Epicoco, Nicola and Dotoli, Mariagrazia}, title = {Modeling, estimation, and analysis of COVID-19 secondary waves: The Case of the Italian Country}, year = {2021}, journal = {2021 29th Mediterranean Conference on Control and Automation, MED 2021}, pages = {794 – 800}, doi = {10.1109/MED51440.2021.9480319}, url = {https://www.scopus.com/inward/record.uri?eid=2-s2.0-85113602900&doi=10.1109%2fMED51440.2021.9480319&partnerID=40&md5=980feaa724975719c46c214ad1dcbfed}, abstract = {The recent trends of the COVID-19 research have been devoted to disease transmission modeling, with the aim of investigating the effects of different mitigation strategies mainly through scenario-based simulations. In this context we propose a novel non-linear time-varying model that effectively supports policy-makers in predicting and analyzing the dynamics of COVID-19 secondary waves. Specifically, this paper proposes an accurate SIRUCQTHE epidemiological model to get reliable predictions on the pandemic dynamics. Differently from the related literature, in the fitting phase, we make use of the google mobility reports to identify and predict the evolution of the infection rate. The effectiveness of the presented method is tested on the network of Italian regions. First, we describe the Italian epidemiological scenario in the COVID-19 second wave of contagions, showing the raw data available for the Italian scenario and discussing the main assumptions on the system parameters. Then, we present the different steps of the procedure used for the dynamical fitting of the SIRUCQTHE model. Finally, we compare the estimation results with the real data on the COVID-19 secondary waves in Italy. Provided the availability of reliable data to calibrate the model in heterogeneous scenarios, the proposed approach can be easily extended to cope with other scenarios. © 2021 IEEE.}, author_keywords = {COVID-19; Dynamical fitting; Estimation; Identification; Pandemic modeling}, keywords = {Forecasting; Disease transmission; Epidemiological modeling; Estimation results; Infection rates; Italian regions; Mitigation strategy; Predicting and analyzing; Scenario-based simulations; Shear waves}, type = {Conference paper}, publication_stage = {Final}, source = {Scopus}, note = {Cited by: 2} }
- Scarabaggio, P., Carli, R., Cavone, G., Epicoco, N. & Dotoli, M. (2021) Modeling, Estimation, and Optimal Control of Anti-COVID-19 Multi-dose Vaccine Administration IN IEEE International Conference on Automation Science and Engineering., 990 – 995. doi:10.1109/CASE49439.2021.9551418
[BibTeX] [Abstract] [Download PDF]The recent trends of the COVID-19 research are being devoted to disease transmission modeling in presence of vaccinated individuals, while the emerging needs are being focused on developing effective strategies for the optimal distribution of vaccine between population. In this context, we propose a novel non-linear time-varying model that effectively supports policy-makers in predicting and analyzing the dynamics of COVID-19 when partially and fully immune individuals are included in the population. Differently from the related literature, where the common strategies typically rely on the prioritization of the different classes of individuals, we propose a novel Model Predictive Control approach to optimally control the multi-dose vaccine administration in the case the available number of doses is not sufficient to cover the whole population. Focusing on the minimization of the expected number of deaths, the approach discriminates between the number of first and second doses. We calibrate the model on the Israeli scenario using real data and we estimate the impact of the vaccine administration on the virus dynamics. Lastly, we assess the impact of the first dose of the Pfizer’s vaccine confirming the results of clinical tests. © 2021 IEEE.
@CONFERENCE{Scarabaggio2021990, author = {Scarabaggio, Paolo and Carli, Raffaele and Cavone, Graziana and Epicoco, Nicola and Dotoli, Mariagrazia}, title = {Modeling, Estimation, and Optimal Control of Anti-COVID-19 Multi-dose Vaccine Administration}, year = {2021}, journal = {IEEE International Conference on Automation Science and Engineering}, volume = {2021-August}, pages = {990 – 995}, doi = {10.1109/CASE49439.2021.9551418}, url = {https://www.scopus.com/inward/record.uri?eid=2-s2.0-85117035432&doi=10.1109%2fCASE49439.2021.9551418&partnerID=40&md5=489bcdcd710fb127ad522dbebe163265}, abstract = {The recent trends of the COVID-19 research are being devoted to disease transmission modeling in presence of vaccinated individuals, while the emerging needs are being focused on developing effective strategies for the optimal distribution of vaccine between population. In this context, we propose a novel non-linear time-varying model that effectively supports policy-makers in predicting and analyzing the dynamics of COVID-19 when partially and fully immune individuals are included in the population. Differently from the related literature, where the common strategies typically rely on the prioritization of the different classes of individuals, we propose a novel Model Predictive Control approach to optimally control the multi-dose vaccine administration in the case the available number of doses is not sufficient to cover the whole population. Focusing on the minimization of the expected number of deaths, the approach discriminates between the number of first and second doses. We calibrate the model on the Israeli scenario using real data and we estimate the impact of the vaccine administration on the virus dynamics. Lastly, we assess the impact of the first dose of the Pfizer's vaccine confirming the results of clinical tests. © 2021 IEEE.}, author_keywords = {COVID-19; model predictive control; pandemic modeling; vaccine; vaccine distribution}, keywords = {Model predictive control; Viruses; COVID-19; Disease transmission; Estimation controls; Model estimation; Model-predictive control; Modelling controls; Optimal controls; Pandemic modeling; Recent trends; Vaccine distributions; Vaccines}, type = {Conference paper}, publication_stage = {Final}, source = {Scopus}, note = {Cited by: 7; All Open Access, Bronze Open Access, Green Open Access} }
2020
- Scarabaggio, P., Carli, R. & Dotoli, M. (2020) A fast and effective algorithm for influence maximization in large-scale independent cascade networks IN 7th International Conference on Control, Decision and Information Technologies, CoDIT 2020., 639 – 644. doi:10.1109/CoDIT49905.2020.9263914
[BibTeX] [Abstract] [Download PDF]A characteristic of social networks is the ability to quickly spread information between a large group of people. The widespread use of online social networks (e.g., Facebook) increases the interest of researchers on how influence propagates through these networks. One of the most important research issues in this field is the so-called influence maximization problem, which essentially consists in selecting the most influential users (i.e., those who are able to maximize the spread of influence through the social network). Due to its practical importance in various applications (e.g., viral marketing), such a problem has been studied in several variants. Nevertheless, the current open challenge in the resolution of the influence maximization problem still concerns achieving a good trade-off between accuracy and computational time. In this context, based on independent cascade modeling of social networks, we propose a novel low-complexity and highly accurate algorithm for selecting an initial group of nodes to maximize the spread of influence in large-scale networks. In particular, the key idea consists in iteratively removing the overlap of influence spread induced by different seed nodes. The application to several numerical experiments based on real datasets proves that the proposed algorithm effectively finds practical near-optimal solutions of the addressed influence maximization problem in a computationally efficient fashion. Finally, the comparison with the state of the art algorithms demonstrates that in large scale scenarios the proposed approach shows higher performance in terms of influence spread and running time. © 2020 IEEE.
@CONFERENCE{Scarabaggio2020639, author = {Scarabaggio, Paolo and Carli, Raffaele and Dotoli, Mariagrazia}, title = {A fast and effective algorithm for influence maximization in large-scale independent cascade networks}, year = {2020}, journal = {7th International Conference on Control, Decision and Information Technologies, CoDIT 2020}, pages = {639 – 644}, doi = {10.1109/CoDIT49905.2020.9263914}, url = {https://www.scopus.com/inward/record.uri?eid=2-s2.0-85098236199&doi=10.1109%2fCoDIT49905.2020.9263914&partnerID=40&md5=003f7d44f921ecd0bf436dacb2d3e136}, abstract = {A characteristic of social networks is the ability to quickly spread information between a large group of people. The widespread use of online social networks (e.g., Facebook) increases the interest of researchers on how influence propagates through these networks. One of the most important research issues in this field is the so-called influence maximization problem, which essentially consists in selecting the most influential users (i.e., those who are able to maximize the spread of influence through the social network). Due to its practical importance in various applications (e.g., viral marketing), such a problem has been studied in several variants. Nevertheless, the current open challenge in the resolution of the influence maximization problem still concerns achieving a good trade-off between accuracy and computational time. In this context, based on independent cascade modeling of social networks, we propose a novel low-complexity and highly accurate algorithm for selecting an initial group of nodes to maximize the spread of influence in large-scale networks. In particular, the key idea consists in iteratively removing the overlap of influence spread induced by different seed nodes. The application to several numerical experiments based on real datasets proves that the proposed algorithm effectively finds practical near-optimal solutions of the addressed influence maximization problem in a computationally efficient fashion. Finally, the comparison with the state of the art algorithms demonstrates that in large scale scenarios the proposed approach shows higher performance in terms of influence spread and running time. © 2020 IEEE.}, keywords = {Economic and social effects; Iterative methods; Computationally efficient; Influence maximizations; Most influential users; Near-optimal solutions; Numerical experiments; On-line social networks; Practical importance; State-of-the-art algorithms; Social networking (online)}, type = {Conference paper}, publication_stage = {Final}, source = {Scopus}, note = {Cited by: 1} }
- Carli, R., Cavone, G., Epicoco, N., Scarabaggio, P. & Dotoli, M. (2020) Model predictive control to mitigate the COVID-19 outbreak in a multi-region scenario. IN Annual Reviews in Control, 50.373 – 393. doi:10.1016/j.arcontrol.2020.09.005
[BibTeX] [Abstract] [Download PDF]The COVID-19 outbreak is deeply influencing the global social and economic framework, due to restrictive measures adopted worldwide by governments to counteract the pandemic contagion. In multi-region areas such as Italy, where the contagion peak has been reached, it is crucial to find targeted and coordinated optimal exit and restarting strategies on a regional basis to effectively cope with possible onset of further epidemic waves, while efficiently returning the economic activities to their standard level of intensity. Differently from the related literature, where modeling and controlling the pandemic contagion is typically addressed on a national basis, this paper proposes an optimal control approach that supports governments in defining the most effective strategies to be adopted during post-lockdown mitigation phases in a multi-region scenario. Based on the joint use of a non-linear Model Predictive Control scheme and a modified Susceptible-Infected-Recovered (SIR)-based epidemiological model, the approach is aimed at minimizing the cost of the so-called non-pharmaceutical interventions (that is, mitigation strategies), while ensuring that the capacity of the network of regional healthcare systems is not violated. In addition, the proposed approach supports policy makers in taking targeted intervention decisions on different regions by an integrated and structured model, thus both respecting the specific regional health systems characteristics and improving the system-wide performance by avoiding uncoordinated actions of the regions. The methodology is tested on the COVID-19 outbreak data related to the network of Italian regions, showing its effectiveness in properly supporting the definition of effective regional strategies for managing the COVID-19 diffusion. © 2020 Elsevier Ltd
@ARTICLE{Carli2020373, author = {Carli, Raffaele and Cavone, Graziana and Epicoco, Nicola and Scarabaggio, Paolo and Dotoli, Mariagrazia}, title = {Model predictive control to mitigate the COVID-19 outbreak in a multi-region scenario}, year = {2020}, journal = {Annual Reviews in Control}, volume = {50}, pages = {373 – 393}, doi = {10.1016/j.arcontrol.2020.09.005}, url = {https://www.scopus.com/inward/record.uri?eid=2-s2.0-85097753585&doi=10.1016%2fj.arcontrol.2020.09.005&partnerID=40&md5=adce49e71a999948867e93de3ae2e142}, abstract = {The COVID-19 outbreak is deeply influencing the global social and economic framework, due to restrictive measures adopted worldwide by governments to counteract the pandemic contagion. In multi-region areas such as Italy, where the contagion peak has been reached, it is crucial to find targeted and coordinated optimal exit and restarting strategies on a regional basis to effectively cope with possible onset of further epidemic waves, while efficiently returning the economic activities to their standard level of intensity. Differently from the related literature, where modeling and controlling the pandemic contagion is typically addressed on a national basis, this paper proposes an optimal control approach that supports governments in defining the most effective strategies to be adopted during post-lockdown mitigation phases in a multi-region scenario. Based on the joint use of a non-linear Model Predictive Control scheme and a modified Susceptible-Infected-Recovered (SIR)-based epidemiological model, the approach is aimed at minimizing the cost of the so-called non-pharmaceutical interventions (that is, mitigation strategies), while ensuring that the capacity of the network of regional healthcare systems is not violated. In addition, the proposed approach supports policy makers in taking targeted intervention decisions on different regions by an integrated and structured model, thus both respecting the specific regional health systems characteristics and improving the system-wide performance by avoiding uncoordinated actions of the regions. The methodology is tested on the COVID-19 outbreak data related to the network of Italian regions, showing its effectiveness in properly supporting the definition of effective regional strategies for managing the COVID-19 diffusion. © 2020 Elsevier Ltd}, author_keywords = {COVID-19; Epidemic control; MPC; Multi-region SIR model; Pandemic modeling; Post-lockdown mitigation strategies; SIR model}, keywords = {Economics; Economic activities; Economic framework; Epidemiological modeling; Health-care system; Mitigation strategy; Non-pharmaceutical interventions; Nonlinear model predictive control; Optimal controls; Model predictive control}, type = {Article}, publication_stage = {Final}, source = {Scopus}, note = {Cited by: 76; All Open Access, Green Open Access} }
- Scarabaggio, P., Carli, R., Cavone, G. & Dotoli, M. (2020) Smart control strategies for primary frequency regulation through electric vehicles: A battery degradation perspective. IN Energies, 13.. doi:10.3390/en13174586
[BibTeX] [Abstract] [Download PDF]Nowadays, due to the decreasing use of traditional generators in favor of renewable energy sources, power grids are facing a reduction of system inertia and primary frequency regulation capability. Such an issue is exacerbated by the continuously increasing number of electric vehicles (EVs), which results in enforcing novel approaches in the grid operations management. However, from being an issue, the increase of EVs may turn to be a solution to several power system challenges. In this context, a crucial role is played by the so-called vehicle-to-grid (V2G) mode of operation, which has the potential to provide ancillary services to the power grid, such as peak clipping, load shifting, and frequency regulation. More in detail, EVs have recently started to be effectively used for one of the most traditional frequency regulation approaches: the so-called frequency droop control (FDC). This is a primary frequency regulation, currently obtained by adjusting the active power of generators in the main grid. Because to the decommissioning of traditional power plants, EVs are thus recognized as particularly valuable solutions since they can respond to frequency deviation signals by charging or discharging their batteries. Against this background, we address frequency regulation of a power grid model including loads, traditional generators, and several EVs. The latter independently participate in the grid optimization process providing the grid with ancillary services, namely the FDC. We propose two novel control strategies for the optimal control of the batteries of EVs during the frequency regulation service. On the one hand, the control strategies ensure re-balancing the power and stabilizing the frequency of the main grid. On the other hand, the approaches are able to satisfy different types of needs of EVs during the charging process. Differently from the related literature, where the EVs perspective is generally oriented to achieve the optimal charge level, the proposed approaches aim at minimizing the degradation of battery devices. Finally, the proposed strategies are compared with other state-of-the-art V2G control approaches. The results of numerical experiments using a realistic power grid model show the effectiveness of the proposed strategies under the actual operating conditions. © 2020 by the authors. Licensee MDPI, Basel, Switzerland. This article is an open access article distributed under the terms and conditions of the Creative Commons Attribution (CC BY) license (http://creativecommons.org/licenses/by/4.0/).
@ARTICLE{Scarabaggio2020, author = {Scarabaggio, Paolo and Carli, Raffaele and Cavone, Graziana and Dotoli, Mariagrazia}, title = {Smart control strategies for primary frequency regulation through electric vehicles: A battery degradation perspective}, year = {2020}, journal = {Energies}, volume = {13}, number = {17}, doi = {10.3390/en13174586}, url = {https://www.scopus.com/inward/record.uri?eid=2-s2.0-85090919511&doi=10.3390%2fen13174586&partnerID=40&md5=d7f07f0a819d149b5f1c143b707e731d}, abstract = {Nowadays, due to the decreasing use of traditional generators in favor of renewable energy sources, power grids are facing a reduction of system inertia and primary frequency regulation capability. Such an issue is exacerbated by the continuously increasing number of electric vehicles (EVs), which results in enforcing novel approaches in the grid operations management. However, from being an issue, the increase of EVs may turn to be a solution to several power system challenges. In this context, a crucial role is played by the so-called vehicle-to-grid (V2G) mode of operation, which has the potential to provide ancillary services to the power grid, such as peak clipping, load shifting, and frequency regulation. More in detail, EVs have recently started to be effectively used for one of the most traditional frequency regulation approaches: the so-called frequency droop control (FDC). This is a primary frequency regulation, currently obtained by adjusting the active power of generators in the main grid. Because to the decommissioning of traditional power plants, EVs are thus recognized as particularly valuable solutions since they can respond to frequency deviation signals by charging or discharging their batteries. Against this background, we address frequency regulation of a power grid model including loads, traditional generators, and several EVs. The latter independently participate in the grid optimization process providing the grid with ancillary services, namely the FDC. We propose two novel control strategies for the optimal control of the batteries of EVs during the frequency regulation service. On the one hand, the control strategies ensure re-balancing the power and stabilizing the frequency of the main grid. On the other hand, the approaches are able to satisfy different types of needs of EVs during the charging process. Differently from the related literature, where the EVs perspective is generally oriented to achieve the optimal charge level, the proposed approaches aim at minimizing the degradation of battery devices. Finally, the proposed strategies are compared with other state-of-the-art V2G control approaches. The results of numerical experiments using a realistic power grid model show the effectiveness of the proposed strategies under the actual operating conditions. © 2020 by the authors. Licensee MDPI, Basel, Switzerland. This article is an open access article distributed under the terms and conditions of the Creative Commons Attribution (CC BY) license (http://creativecommons.org/licenses/by/4.0/).}, author_keywords = {Electric vehicle batteries (EVBs); Electric vehicles (EVs); Frequency droop control (FDC); Vehicle-to-grid (V2G)}, keywords = {Automotive batteries; Charging (batteries); Electric control equipment; Electric generators; Electric network topology; Electric power transmission networks; Electric vehicles; Microgrids; Renewable energy resources; Vehicle-to-grid; Electric Vehicles (EVs); Frequency regulation services; Frequency regulations; Numerical experiments; Primary frequency regulation; Renewable energy source; Smart control strategies; Vehicle to Grid (V2G); Electric power system control}, type = {Article}, publication_stage = {Final}, source = {Scopus}, note = {Cited by: 37; All Open Access, Gold Open Access, Green Open Access} }
- Scarabaggio, P., Carli, R., La Scala, M. & Dotoli, M. (2020) Effects of COVID-19 on electricity demand in Northern Italy; [Effetti del COVID-19 sulla domanda di energia elettrica nel Nord Italia]. IN Energia Elettrica, 97.41 – 51.
[BibTeX] [Abstract] [Download PDF]Technical analysis of the effects of the COVID-19 pandemic on electricity demand: the case of Northern Italy. Estimation of the impact of social mobility on electricity consumption. Future challenges and prospects.
@ARTICLE{Scarabaggio202041, author = {Scarabaggio, Paolo and Carli, Raffaele and La Scala, Massimo and Dotoli, Mariagrazia}, title = {Effects of COVID-19 on electricity demand in Northern Italy; [Effetti del COVID-19 sulla domanda di energia elettrica nel Nord Italia]}, year = {2020}, journal = {Energia Elettrica}, volume = {97}, number = {5}, pages = {41 – 51}, url = {https://www.scopus.com/inward/record.uri?eid=2-s2.0-85148942790&partnerID=40&md5=a3679ff0d5aca129d11834c5886fe935}, abstract = {Technical analysis of the effects of the COVID-19 pandemic on electricity demand: the case of Northern Italy. Estimation of the impact of social mobility on electricity consumption. Future challenges and prospects.}, type = {Article}, publication_stage = {Final}, source = {Scopus}, note = {Cited by: 0} }
- Scarabaggio, P., Grammatico, S., Carli, R. & Dotoli, M. (2020) A distributed, rolling-horizon demand side management algorithm under wind power uncertainty IN IFAC-PapersOnLine., 12620 – 12625. doi:10.1016/j.ifacol.2020.12.1830
[BibTeX] [Abstract] [Download PDF]In this paper, we consider a smart grid where users behave selfishly, aiming at minimizing cost in the presence of uncertain wind power availability. We adopt a demand side management (DSM) model, where active users (so-called prosumers) have both private generation and local storage availability. These prosumers participate to the DSM strategy by updating their energy schedule, seeking to minimize their local cost, given their local preferences and the global grid constraints. The energy price is defined as a function of the aggregate load and the wind power availability. We model the resulting problem as a non-cooperative Nash game and propose a semi-decentralized algorithm to compute an equilibrium. To cope with the uncertainty in the wind power, we adopt a rolling-horizon approach, and in addition we use a stochastic optimization technique. We generate several wind power production scenarios from a defined probability density function (PDF), determining an approximate stochastic cost function. Simulations results on a real dataset show that the proposed approach generates lower individual costs compared to a standard expected value approach. Copyright © 2020 The Authors. This is an open access article under the CC BY-NC-ND license
@CONFERENCE{Scarabaggio202012620, author = {Scarabaggio, Paolo and Grammatico, Sergio and Carli, Raffaele and Dotoli, Mariagrazia}, title = {A distributed, rolling-horizon demand side management algorithm under wind power uncertainty}, year = {2020}, journal = {IFAC-PapersOnLine}, volume = {53}, number = {2}, pages = {12620 – 12625}, doi = {10.1016/j.ifacol.2020.12.1830}, url = {https://www.scopus.com/inward/record.uri?eid=2-s2.0-85105077171&doi=10.1016%2fj.ifacol.2020.12.1830&partnerID=40&md5=ee3d9dd2c0187078aed713ccc87a026b}, abstract = {In this paper, we consider a smart grid where users behave selfishly, aiming at minimizing cost in the presence of uncertain wind power availability. We adopt a demand side management (DSM) model, where active users (so-called prosumers) have both private generation and local storage availability. These prosumers participate to the DSM strategy by updating their energy schedule, seeking to minimize their local cost, given their local preferences and the global grid constraints. The energy price is defined as a function of the aggregate load and the wind power availability. We model the resulting problem as a non-cooperative Nash game and propose a semi-decentralized algorithm to compute an equilibrium. To cope with the uncertainty in the wind power, we adopt a rolling-horizon approach, and in addition we use a stochastic optimization technique. We generate several wind power production scenarios from a defined probability density function (PDF), determining an approximate stochastic cost function. Simulations results on a real dataset show that the proposed approach generates lower individual costs compared to a standard expected value approach. Copyright © 2020 The Authors. This is an open access article under the CC BY-NC-ND license}, author_keywords = {Demand side management; Sample average approximation; Smart grid; Stochastic optimization}, keywords = {Approximation algorithms; Cost functions; Demand side management; Electric power transmission networks; Electric utilities; Optimization; Probability density function; Stochastic systems; Wind power; Energy; Management Model; Management strategies; Minimizing costs; Rolling horizon; Sample average approximation; Smart grid; Stochastic optimizations; Uncertainty; Wind power availability; Smart power grids}, type = {Conference paper}, publication_stage = {Final}, source = {Scopus}, note = {Cited by: 3; All Open Access, Gold Open Access} }
- Scarabaggio, P., La Scala, M., Carli, R. & Dotoli, M. (2020) Analyzing the Effects of COVID-19 Pandemic on the Energy Demand: The Case of Northern Italy IN 12th AEIT International Annual Conference, AEIT 2020.. doi:10.23919/AEIT50178.2020.9241136
[BibTeX] [Abstract] [Download PDF]The COVID-19 crisis is profoundly influencing the global economic framework due to restrictive measures adopted by governments worldwide. Finding real-time data to correctly quantify this impact is very significant but not as straightforward. Nevertheless, an analysis of the power demand profiles provides insight into the overall economic trends. To accurately assess the change in energy consumption patterns, in this work we employ a multi-layer feed-forward neural network that calculates an estimation of the aggregated power demand in the north of Italy, (i.e, in one of the European areas that were most affected by the pandemics) in the absence of the COVID-19 emergency. After assessing the forecasting model reliability, we compare the estimation with the ground truth data to quantify the variation in power consumption. Moreover, we correlate this variation with the change in mobility behaviors during the lockdown period by employing the Google mobility report data. From this unexpected and unprecedented situation, we obtain some intuition regarding the power system macro-structure and its relation with the overall people’s mobility. © 2020 AEIT.
@CONFERENCE{Scarabaggio2020, author = {Scarabaggio, Paolo and La Scala, Massimo and Carli, Raffaele and Dotoli, Mariagrazia}, title = {Analyzing the Effects of COVID-19 Pandemic on the Energy Demand: The Case of Northern Italy}, year = {2020}, journal = {12th AEIT International Annual Conference, AEIT 2020}, doi = {10.23919/AEIT50178.2020.9241136}, url = {https://www.scopus.com/inward/record.uri?eid=2-s2.0-85097170993&doi=10.23919%2fAEIT50178.2020.9241136&partnerID=40&md5=a3abf95802bb66dc9a2d8715976cc126}, abstract = {The COVID-19 crisis is profoundly influencing the global economic framework due to restrictive measures adopted by governments worldwide. Finding real-time data to correctly quantify this impact is very significant but not as straightforward. Nevertheless, an analysis of the power demand profiles provides insight into the overall economic trends. To accurately assess the change in energy consumption patterns, in this work we employ a multi-layer feed-forward neural network that calculates an estimation of the aggregated power demand in the north of Italy, (i.e, in one of the European areas that were most affected by the pandemics) in the absence of the COVID-19 emergency. After assessing the forecasting model reliability, we compare the estimation with the ground truth data to quantify the variation in power consumption. Moreover, we correlate this variation with the change in mobility behaviors during the lockdown period by employing the Google mobility report data. From this unexpected and unprecedented situation, we obtain some intuition regarding the power system macro-structure and its relation with the overall people's mobility. © 2020 AEIT.}, author_keywords = {COVID-19; Lockdown; Machine learning; Neural networks; Power systems}, keywords = {Electric power utilization; Energy utilization; Feedforward neural networks; Economic trends; Forecasting modeling; Global economics; Ground truth data; Macrostructures; Mobility behavior; Multilayer feedforward neural networks; Northern Italy; Multilayer neural networks}, type = {Conference paper}, publication_stage = {Final}, source = {Scopus}, note = {Cited by: 14; All Open Access, Green Open Access} }
- Scarabaggio, P., Carli, R. & Dotoli, M. (2020) A game-theoretic control approach for the optimal energy storage under power flow constraints in distribution networks IN IEEE International Conference on Automation Science and Engineering., 1281 – 1286. doi:10.1109/CASE48305.2020.9216800
[BibTeX] [Abstract] [Download PDF]Traditionally, the management of power distribution networks relies on the centralized implementation of the optimal power flow and, in particular, the minimization of the generation cost and transmission losses. Nevertheless, the increasing penetration of both renewable energy sources and independent players such as ancillary service providers in modern networks have made this centralized framework inadequate. Against this background, we propose a noncooperative game-theoretic framework for optimally controlling energy storage systems (ESSs) in power distribution networks. Specifically, in this paper we address a power grid model that comprehends traditional loads, distributed generation sources and several independent energy storage providers, each owning an individual ESS. Through a rolling-horizon approach, the latter participate in the grid optimization process, aiming both at increasing the penetration of distributed generation and leveling the power injection from the transmission grid. Our framework incorporates not only economic factors but also grid stability aspects, including the power flow constraints. The paper fully describes the distribution grid model as well as the underlying market hypotheses and policies needed to force the energy storage providers to find a feasible equilibrium for the network. Numerical experiments based on the IEEE 33-bus system confirm the effectiveness and resiliency of the proposed framework. © 2020 IEEE.
@CONFERENCE{Scarabaggio20201281, author = {Scarabaggio, Paolo and Carli, Raffaele and Dotoli, Mariagrazia}, title = {A game-theoretic control approach for the optimal energy storage under power flow constraints in distribution networks}, year = {2020}, journal = {IEEE International Conference on Automation Science and Engineering}, volume = {2020-August}, pages = {1281 – 1286}, doi = {10.1109/CASE48305.2020.9216800}, url = {https://www.scopus.com/inward/record.uri?eid=2-s2.0-85094128798&doi=10.1109%2fCASE48305.2020.9216800&partnerID=40&md5=e4802c482f96ac90afd6c7a2c7f2b199}, abstract = {Traditionally, the management of power distribution networks relies on the centralized implementation of the optimal power flow and, in particular, the minimization of the generation cost and transmission losses. Nevertheless, the increasing penetration of both renewable energy sources and independent players such as ancillary service providers in modern networks have made this centralized framework inadequate. Against this background, we propose a noncooperative game-theoretic framework for optimally controlling energy storage systems (ESSs) in power distribution networks. Specifically, in this paper we address a power grid model that comprehends traditional loads, distributed generation sources and several independent energy storage providers, each owning an individual ESS. Through a rolling-horizon approach, the latter participate in the grid optimization process, aiming both at increasing the penetration of distributed generation and leveling the power injection from the transmission grid. Our framework incorporates not only economic factors but also grid stability aspects, including the power flow constraints. The paper fully describes the distribution grid model as well as the underlying market hypotheses and policies needed to force the energy storage providers to find a feasible equilibrium for the network. Numerical experiments based on the IEEE 33-bus system confirm the effectiveness and resiliency of the proposed framework. © 2020 IEEE.}, keywords = {Data storage equipment; Distributed power generation; Electric load flow; Electric network analysis; Electric power system economics; Electric power transmission; Energy storage; Game theory; Renewable energy resources; Storage as a service (STaaS); Distributed generation source; Energy Storage Systems (ESSs); Noncooperative game; Numerical experiments; Optimal power flows; Power distribution network; Renewable energy source; Transmission grids; Electric power transmission networks}, type = {Conference paper}, publication_stage = {Final}, source = {Scopus}, note = {Cited by: 11} }
- Carli, R., Cavone, G., Epicoco, N., Di Ferdinando, M., Scarabaggio, P. & Dotoli, M. (2020) Consensus-Based Algorithms for Controlling Swarms of Unmanned Aerial Vehicles. IN Lecture Notes in Computer Science (including subseries Lecture Notes in Artificial Intelligence and Lecture Notes in Bioinformatics), 12338 LNCS.84 – 99. doi:10.1007/978-3-030-61746-2_7
[BibTeX] [Abstract] [Download PDF]Multiple Unmanned Aerial Vehicles (multi-UAVs) applications are recently growing in several fields, ranging from military and rescue missions, remote sensing, and environmental surveillance, to meteorology, logistics, and farming. Overcoming the limitations on battery lifespan and on-board processor capabilities, the coordinated use of multi-UAVs is indeed more suitable than employing a single UAV in certain tasks. Hence, the research on swarm of UAVs is receiving increasing attention, including multidisciplinary aspects, such as coordination, aggregation, network communication, path planning, information sensing, and data fusion. The focus of this paper is on defining novel control strategies for the deployment of multi-UAV systems in a distributed time-varying set-up, where UAVs rely on local communication and computation. In particular, modeling the dynamics of each UAV by a discrete-time integrator, we analyze the main swarm intelligence strategies, namely flight formation, swarm tracking, and social foraging. First, we define a distributed control strategy for steering the agents of the swarm towards a collection point. Then, we cope with the formation control, defining a procedure to arrange agents in a family of geometric formations, where the distance between each pair of UAVs is predefined. Subsequently, we focus on swarm tracking, defining a distributed mechanism based on the so-called leader-following consensus to move the entire swarm in accordance with a predefined trajectory. Moreover, we define a social foraging strategy that allows agents to avoid obstacles, by imposing on-line a time-varying formation pattern. Finally, through numerical simulations we show the effectiveness of the proposed algorithms. © 2020, Springer Nature Switzerland AG.
@ARTICLE{Carli202084, author = {Carli, Raffaele and Cavone, Graziana and Epicoco, Nicola and Di Ferdinando, Mario and Scarabaggio, Paolo and Dotoli, Mariagrazia}, title = {Consensus-Based Algorithms for Controlling Swarms of Unmanned Aerial Vehicles}, year = {2020}, journal = {Lecture Notes in Computer Science (including subseries Lecture Notes in Artificial Intelligence and Lecture Notes in Bioinformatics)}, volume = {12338 LNCS}, pages = {84 – 99}, doi = {10.1007/978-3-030-61746-2_7}, url = {https://www.scopus.com/inward/record.uri?eid=2-s2.0-85093852465&doi=10.1007%2f978-3-030-61746-2_7&partnerID=40&md5=1c7da6000e4015880227c1eafe608f20}, abstract = {Multiple Unmanned Aerial Vehicles (multi-UAVs) applications are recently growing in several fields, ranging from military and rescue missions, remote sensing, and environmental surveillance, to meteorology, logistics, and farming. Overcoming the limitations on battery lifespan and on-board processor capabilities, the coordinated use of multi-UAVs is indeed more suitable than employing a single UAV in certain tasks. Hence, the research on swarm of UAVs is receiving increasing attention, including multidisciplinary aspects, such as coordination, aggregation, network communication, path planning, information sensing, and data fusion. The focus of this paper is on defining novel control strategies for the deployment of multi-UAV systems in a distributed time-varying set-up, where UAVs rely on local communication and computation. In particular, modeling the dynamics of each UAV by a discrete-time integrator, we analyze the main swarm intelligence strategies, namely flight formation, swarm tracking, and social foraging. First, we define a distributed control strategy for steering the agents of the swarm towards a collection point. Then, we cope with the formation control, defining a procedure to arrange agents in a family of geometric formations, where the distance between each pair of UAVs is predefined. Subsequently, we focus on swarm tracking, defining a distributed mechanism based on the so-called leader-following consensus to move the entire swarm in accordance with a predefined trajectory. Moreover, we define a social foraging strategy that allows agents to avoid obstacles, by imposing on-line a time-varying formation pattern. Finally, through numerical simulations we show the effectiveness of the proposed algorithms. © 2020, Springer Nature Switzerland AG.}, author_keywords = {Swarm intelligence; Trajectory control; Unmanned Aerial Vehicles}, keywords = {Aircraft detection; Antennas; Data fusion; Distributed parameter control systems; Military applications; Military vehicles; Remote sensing; Unmanned aerial vehicles (UAV); Control strategies; Discrete-time integrators; Distributed control strategy; Environmental surveillance; Local communications; Network communications; Onboard processors; Time-varying formations; Mobile ad hoc networks}, type = {Conference paper}, publication_stage = {Final}, source = {Scopus}, note = {Cited by: 19} }
2019
- Carli, R., Cavone, G., Dotoli, M., Epicoco, N. & Scarabaggio, P. (2019) Model predictive control for thermal comfort optimization in building energy management systems IN Conference Proceedings – IEEE International Conference on Systems, Man and Cybernetics., 2608 – 2613. doi:10.1109/SMC.2019.8914489
[BibTeX] [Abstract] [Download PDF]Model Predictive Control (MPC) has recently gained special attention to efficiently regulate Heating, Ventilation and Air Conditioning (HVAC) systems of buildings, since it explicitly allows energy savings while maintaining thermal comfort criteria. In this paper we propose a MPC algorithm for the on-line optimization of both the indoor thermal comfort and the related energy consumption of buildings. We use Fanger’s Predicted Mean Vote (PMV) as thermal comfort index, while to predict the energy performance of the building, we adopt a simplified thermal model. This allows computing optimal control actions by defining and solving a tractable non-linear optimization problem that incorporates the PMV index into the MPC cost function in addition to a term accounting for energy saving. The proposed MPC approach is implemented on a building automation system deployed in an office building located at the Polytechnic of Bari (Italy). Several on-field tests are performed to assess the applicability and efficacy of the control algorithm in a real environment against classical thermal comfort control approach based on the use of thermostats. © 2019 IEEE.
@CONFERENCE{Carli20192608, author = {Carli, Raffaele and Cavone, Graziana and Dotoli, Mariagrazia and Epicoco, Nicola and Scarabaggio, Paolo}, title = {Model predictive control for thermal comfort optimization in building energy management systems}, year = {2019}, journal = {Conference Proceedings - IEEE International Conference on Systems, Man and Cybernetics}, volume = {2019-October}, pages = {2608 – 2613}, doi = {10.1109/SMC.2019.8914489}, url = {https://www.scopus.com/inward/record.uri?eid=2-s2.0-85076778873&doi=10.1109%2fSMC.2019.8914489&partnerID=40&md5=3c982fb93adbcfb5202b48b60ad0f22d}, abstract = {Model Predictive Control (MPC) has recently gained special attention to efficiently regulate Heating, Ventilation and Air Conditioning (HVAC) systems of buildings, since it explicitly allows energy savings while maintaining thermal comfort criteria. In this paper we propose a MPC algorithm for the on-line optimization of both the indoor thermal comfort and the related energy consumption of buildings. We use Fanger's Predicted Mean Vote (PMV) as thermal comfort index, while to predict the energy performance of the building, we adopt a simplified thermal model. This allows computing optimal control actions by defining and solving a tractable non-linear optimization problem that incorporates the PMV index into the MPC cost function in addition to a term accounting for energy saving. The proposed MPC approach is implemented on a building automation system deployed in an office building located at the Polytechnic of Bari (Italy). Several on-field tests are performed to assess the applicability and efficacy of the control algorithm in a real environment against classical thermal comfort control approach based on the use of thermostats. © 2019 IEEE.}, keywords = {Air conditioning; Automation; Cost functions; Energy conservation; Energy management systems; Energy utilization; Intelligent buildings; Nonlinear programming; Office buildings; Predictive control systems; Thermal comfort; Building automation systems; Energy performance; Indoor thermal comfort; Non-linear optimization problems; Online optimization; Predicted mean vote; Thermal comfort control; Thermal comfort index; Model predictive control}, type = {Conference paper}, publication_stage = {Final}, source = {Scopus}, note = {Cited by: 20} }